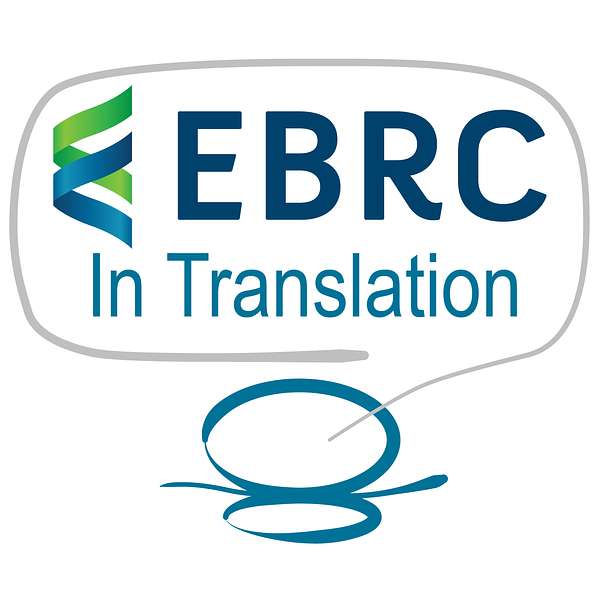
EBRC In Translation
EBRC In Translation
27. Non-Model Careers and Open-Source Biology w/ Prachee Avasthi
In this episode, we interview Dr. Prachee Avasthi, Co-founder and CSO of Arcadia Science and previous Associate Professor of Biochemistry and Cell Biology at Dartmouth. We talk with Prachee about her many layers of experiments, from mining new biological tools to building new organizational structures for open science. We also talk with her about non-canonical career paths, science publishing models, and much more!
For more information about EBRC:
Visit our website at ebrc.org. If you are interested in getting involved with the EBRC Student and Postdoc Association, fill out a membership application for graduate students and postdocs or for undergraduates and join today!
Transcription:
Episode transcripts are the unedited output from Whisper and likely contain errors.
Hello, and welcome back to EBRC in Translation. We're a group of graduate students and postdocs working to bring you conversations with members of the engineering biology community. I'm Andrew Hunt, a postdoc in the Baker Lab at the University of Washington. And I'm Will Groobie, a PhD candidate in the Mendoza and DePapo labs at the University of Chicago. Today we are joined by Dr. Prachi Avashti. Prachi is the co-founder and CSO of Arcadia Science, a company working to discover new biological tools to solve societal challenges. Previously, she was an associate professor of biochemistry and cell biology in the Giselle School of Medicine at Dartmouth. She is also the president of ASAPbio. And thank you so much for joining us today, Prachi. Yeah, thanks so much for having me. I'm really excited to be here. So to get us started, could you tell us a bit about your journey from doing a neuroscience PhD to founding Arcadia and maybe a bit about some of the key experiences that led you down the path you've taken? Yeah, absolutely. So I can say confidently that this was not part of my plan. And I think one of the best things that I've learned in the last few years is that, you know, it's sort of not that imperative that we know what we're going to be when we grow up. I still don't know what I'm going to be when I grow up. So yeah, and I think that was with a pretty linear path to sort of an academic career that, you know, relative to, you know, others. So I did my PhD in neuroscience and at the University of Utah. And even in graduate school, I had done an internship at Genentech right before I graduated. And I remember at the time thinking like, this was awesome. Like, I loved it. I thought it was an electric atmosphere. I thought the people very smart. I thought it was extremely like an informative learning experience for me. I think it really impacted how I ran my own lab. And, you know, when it came to, you know, resource allocation, things like that, you know, just thinking about scientific strategy, I think I learned a lot from that experience and still and truly loved it and still came away thinking that, you know, oh, yeah, you know, the thing that you do and the thing that I'm going to do is start an academic lab. And so I, you know, went on from graduate school to, you know, move, start working on a simple model system. So I was working largely as a grad student in most photoreceptors. So mammalian system that felt pretty like slow to me compared to all the things that I was reading in the literature that really got to some of the mechanistic details of things I wanted to investigate. And all of that was done in a really simple unicellular organism. So that's the thing that I wanted to move into. So, you know, stronger genetic tools, easier biochemistry in this model algo, which is what I ended up working on in my postdoc. And so I came to San Francisco, did my postdoc at ECSF, working largely to uncover like mechanistic details of this organelle biogenesis that I was studying. So of this organelle called the psyllium, which is really a two way antenna that is on virtually all cells of the human body and conserved across the tree of life. But so simple and conserved that you could really study this in a unicellular green alga. So really interesting biology got a lot that I wanted out of it and then went on to that traditional path. And I started my own lab about 2015 at the University of Kansas Medical Center in Kansas City. And I stayed there for five years. So I went there, got tenure there, and then decided to move my lab to Dartmouth, which is what happened at the beginning of the pandemic. So, you know, upped and moved my entire lab to, you know, a place that I had never been before. My family had never been before. It was kind of a big leap of faith in that moment. It was interesting, it was kind of remote up there. So things were a lot more open during the pandemic than they were in other places. So we were not that hampered and getting things off the ground, ready to sort of take in new students, you know, get everything wrapped up. And about, you know, less than a year later, I was put in touch with a mutual friend, someone, a colleague of mine at ASAPBio. So I'm the president of ASAPBio, this, you know, organization that works on innovation and life sciences communication, and really a preprint advocacy organization. And the vice president of that is a guy named Jamie Frazier, who is a professor at UCSF. You know, I had told him that I was really hungry for a new project, which is kind of funny, given that I just sort of moved my family in my lab, and was just getting things up and running again. And he said, Oh, well, you know, I was gonna wait to tell you this, but a friend of mine, Simei, has been thinking about investing in this organization, thinking about, you know, leveraging non-model systems, and really wants there to be an open science component. And that is something that I've been working on for a long time, you know, really thinking through a variety of different organizations on different boards, different journals, of how to reimagine how we get ourselves out of this mess of going after the most inefficient possible way of communicating our science, inefficient and expensive way to communicate our science that doesn't really achieve its goals. He said, you know, you should talk to each other. And so I think contrary to what many people thinks, my co founder and I didn't know each other beforehand, I hear that's quite rare, or I hear it's quite rare for such, you know, situations to really last, given that you don't have a strong, you know, history or bonds over other things besides, you know, launching an org. But we very much didn't know each other beforehand, and really started thinking about what it would look like to, you know, generate an engine for basic science that really was efficient at coming to really useful outcomes, right? So we are all familiar with mining biology to come up with new innovations. So CRISPR, GFP, PCR, these things all came from, you know, a diverse set of biology. But, you know, getting from that to something that becomes, you know, very useful to a lot of people can take decades, hundreds of scientists, and a whole lot of luck. And the question we had was, can we actually do this in a rational, principled way? Can we actually reduce that to the amount of time that's actually feasible, you know, within an organization? Can we find some sort of roadmap to do it, you know? And we know that, you know, trying to figure those things out is like a risky endeavor, but we had sort of a lot of runway to be able to try and figure that out. And just thought it was, you know, important and worth trying. So we started thinking about what it could be, who would be involved, what's the structure of the organization, is it, you know, what's the, you know, communication model, like all of the things that you can think about, what's the business plan, like all of these things, sort of writing down a ton of documents, trying to really flush it out, and started brainstorming with others. And eventually, we got to the point where we showed it to a room of people to say, like, here's what we've been thinking. And in the end, we sort of said, like, are we gonna just talk about it? Are we gonna do it? And we sort of made a plan that we would, you know, we would get our, you know, this group of people together and just go for it, you know, and just like, try to make it happen. And in the end, five seconds after making that plan, we were like, you know what, screw it, like, you and I will just do it. Let's just like, give people a place to land. You make something exist. And by virtue of that, try to lure others that might be like minded and want to help us build something like it. So yeah, it was sort of not the plan and never something I thought I would do. And, you know, but it was definitely, I would say, a hundred percent pull rather than push. To me, it felt like, you know, an opportunity to try and do something very hard that we didn't know if we could do it. That would be a hugely impactful thing if we could try and run the types of experiments and how to run an organization, how to think about, you know, doing efficient basic science, make an organization that's self-sustaining, really push the boundaries of open science and commercialization to test whether, you know, that they aren't really necessarily at odds with one another. Can they be synergistic? Can we accelerate the science and really do better, more rigorous science by doing it out in the open and still preserve our ability to make a self-sustaining organization? That's something that would be really useful and also make it so that other people could try and launch organizations. Like, you don't want to have only people with deep pockets, you know, starting new things. You want more people to try more things. And how do we generate a model that could be useful to other people in starting something new and interesting? And that's something we're really interested in to make sure the impact is always, you know, bigger than our walls. That's great. Yeah, it's such an interesting and unique company. Maybe this is a good time if you could dive deeper into Arcadia's specific mission and some of its operations. We're also curious to hear about unique challenges or opportunities that, you know, you touched briefly on what led you to establish the company, but maybe as you're going from idea to company, how things change or anything unique that came up. Yeah, sometimes, you know, just to get to that part real quick, like sometimes I say we're on, like, the 10,000th iteration of this organization, you know, and it is very, everything's changing all the time. We're always pivoting. We're always trying to figure out new ways to do things. Arcadia's, you know, specific goal is to try and see if we can leverage the biology of diverse organisms, but do so in a way, you know, for, you know, useful outcomes and purposes. You know, can we actually innovate? Can we, you know, solve biological problems and ask better questions through sort of mining the tree of life? Biology has done so many experiments, so many more experiments than we could ever do in a lab, right? And so can we actually rationally mine that space? Can we increase the search space? Can we rationally mine it to more efficiently, you know, traverse biology in ways that actually make it possible for us to get to outcomes faster and in a better way? So that really is something that we're trying to do. I say that as a concept, like, can we mine biology? Can we, you know, use evolution? But, you know, how, right? So that's the thing that we're actually building in-house with our in-house platforms. So we sort of have different arms of the organization, you know, a discovery platform that is our, you know, thesis for how do we go about mining biology using what we, like, data we have. So of course, we want to move outside of this canonical wisdom of, you know, how we've all learned how to do science, right? Which is like, what's been done before? Let's look and see what has been done and find the edges and boundaries of that information and then move past it, right? Like, that's how we've learned to do science, right? But when you think about life, it's so vast, right? And most people have not worked on most things. So if we just looked at what's been done, it's very much looking under the lamp post, right? And so what we want to do is actually expand that out and say, we want to be able to leverage more biology, but it's enormous, right? So we're really starting from a thing that we have more information on, which is what is in the literature in journal articles, right? Which is genomes. We know that we have a lot more genomes than we have of other information. And a lot of it is, you know, not fully mined. We haven't not fully annotated all of these things, but we have data for things outside of what people have worked on. And can we leverage that information to actually unearth some sort of signal in the noise? Can we actually find patterns of evolution that give us some sort of idea that something interesting has taken place in biology, right? So if you, I strongly encourage you to look at our publications on our website, research our kdscience.com, and you can actually read our work on a pub that's called Novel Tree that really allows us to compare gene family evolution with species evolution and do, you know, reconcile those things to identify where there's a gene duplication transfer loss, these sort of different types of evolutionary signals that something is happening and try to map that to outcomes to see what that innovation or novelty is for. So we really are trying to leverage the information we have in order to more agnostically identify sort of signals in the noise for what might be worth pursuing. And then also doing something similar to that using protein structure, actually. So we know that, you know, sometimes I say that, you know, Arcadia is held together by duct tape and alpha fold. Just, it's like completely transformed the way we think about biology and really thinking about that even though when you're looking across the tree of life, you know, sequence is not as well conserved a structure in many cases and really trying to look at protein structural prediction and looking at ways in which we can do structure function mapping and identify novel domains, features of different proteins. So rather than engineering everything from scratch, can we also like leverage what biology has already done that might be already functional and uncover new structure function relationships and, you know, both annotate genes this way, but also identify new solutions that we can hone and re-engineer for useful purposes. So we have sort of a discovery platform that's doing these types of things like building the functionality to try and mine, you know, biology. And then we want to, of course, put that in action. Can we actually use the tools that we're building in-house and make the science that we're doing more efficient? And that's why we have a translation arm that's really just doing a bunch of different projects in-house to say, you know, there's certain ways that you would go about doing the biology normally, but by virtue of leveraging our discovery platform, can they get more efficient at whatever solution they're after? And so we have both of those things going on along with an engineering arm that's really helps to, you know, automate and scale and be the interface between our discovery and translation arms. But that's sort of the structure of Arcadia where we have, you know, really fundamentally a bunch of different sort of North stars, but all working together to see if we can get, you know, more efficient ways to get from mining useful discovery and getting them out into the world. But then we also have separate missions that we think have to be aligned with that. So we are very much an open science organization, really thinking about how we can, you know, again, I think open science is better science, faster, more rigorous. Like, can we actually, by virtue of sharing the information that we're learning for so many things, like the basic science discoveries are going to be way more useful to the world than they're going to be for us to, you know, the fraction of them that are going to be useful for us to commercialize. Right. So a lot of people ask me, how are those, how can you square those two things? How do you actually reconcile open science and commercialization? And we really think that, you know, these things can be synergistic, that if you really wanted to do the best science and fastest science, like I would want to do that in an open way and leverage the capacity and, you know, brilliance of the universe and not just what's within our walls. Right. We really want to get things out there and stress test ourselves and get other people to use the things that we're building, help make it better, tell us how we can make things better for the type of science that they're tackling. And you want to sort of develop those things in real world scenarios for things that people actually use them for. But then, and by virtue of that, we can make our own process better to make it more useful for things that we're spinning out ourselves. So we have, again, a publishing arm that really allows us to share our science early and more often. We've said a long time ago that we're not going to do traditional journal publication. And then, of course, we want to, you know, we are a for-profit company and are doing so primarily with the goal of spinning out our own companies. You know, we start with a project incubated in-house. We, you know, it's very early. This is like pre-Idea stage things that we are starting. So sooner than people would typically invest in them. And then the goal is to actually de-risk it ourselves through our discovery platforms and other ways and try to get it to the point where it could actually spin out to its own entity, something that we have a stake in that could help make us self-sustaining. So that's really the model for how we intend to sort of generate revenue. But again, trying to do that in the most efficient way possible, leveraging our discovery platforms, doing science as openly as we can, and then trying to get to those useful outcomes. Yeah, very cool. I want to try and go back to something you just said, Prachi, which is sort of like balancing the incentives of doing basic research and sort of industry work. And Acadia as an experiment seems really interesting to me because it's sort of like trying to do that in a space that we don't normally see it. And it sounds challenging. So could you delve a little bit more into that, like how you're trying to balance traditional industry incentives and doing basic science? Yeah, I will say that for us, you know, the primary mission is really to figure out how to do this as an organization, our sort of meta science goal of trying to figure out the types of things that a company wouldn't be able to do because they have to make a beeline for profit. Otherwise they're going to run out of money and it's game over, right? And, you know, we have more one way than that. So we have the chance to really figure out what is the right way to do this. And so that is something that is fundamentally important to us. And, you know, we know that in trying to figure these things out, there's going to be tradeoffs, right? You know, there are going to be things where is it possible that we don't capitalize on everything that might have generated revenue? Yes. Like we're prepared to do that to try and stress test it in a way that others might not be able to. Right. So for us, you're not wrong in the sense that we have found that, you know, there are so many different models and ways of doing science. And, you know, for when you're looking for therapeutic outcomes, for example, like having your eye on the prize and really figuring out how to make that happen is extremely important, but that might be something different than what discovery science is actually actually trying to figure out, you know, pathways and pipelines to actually make science more efficient. That needs to be multipurpose and not just fixated on a single goal is maybe more useful. So one of the things that we've learned, so we've, you know, like I said, multiple iterations of this company, we've had it all in wonder umbrella. And then we've now split it off into discovery and engineering and translation, you know, just because that, because the shape of the science might look a little bit different and the goals for the different projects are a little bit different. So for example, we have discovery pilots where the entire purpose is to seed new ways or expand our functionality to mine biology, right? Like that is the goal of those projects. And then we have translation project where the goal is actually to go towards some sort of translational outcome. Right. We found that it was really important to make the goals clear. Otherwise everyone was trying to do everything. It's like, if you say everything's important, then nothing's important. Right. And so it really became a problem of really muddying that goal and making, you know, it's sometimes I make the analogy. Sometimes I say it's like the car seat problem where if you try to make something functional for an infant and, you know, you know, older kid, it's going to be a crappy version for all involved, you know, that you actually want to have something that is like fit for purpose for the thing that you're actually trying to achieve. And so I guess that is something that we have iterated on within the organization is really thinking about, you know, what are our goals? And when you have focused goals, like if your focused goal is to generate a multipurpose pipeline that's going to make the science more efficient, then that needs to be eye on the prize on that thing. And that's what those the people working on that are really focused on that effort and figuring out what the internal and external users might want. And then, you know, and doing this sort of research problems of figuring that out, there are a lot of sort of fundamental biological research problems in how to do that better. You know, there's definitely be scientists we have in house who are like laser focused on, but tell me how this gets to some outcome. You know, tell me how this actually solves this type of, you know, gets us closer to a therapeutic. And, and that is another laser focused goal that has its own constraints that look different, you know. And so I think that bit of separation was something that was like extremely useful for us. It was also extremely useful for the scientists in house to really been where did their strengths were, and that was important learning for us. Great. We had a question about a lot of the model organisms that you guys study and kind of the history you briefly talked about kind of what led to that and your work, your research work that led to this interest in mining other types of biological organisms to find new types of tools. Were there any specific organisms you were interested to study early on in Arcadia that really prompted you to build a company like that? Or are there any fun ones that you would like to highlight? Because looking through the website, it's really great to see just several dozen of cool biological organisms. Oh, man, it's so cool. Okay, so the funny thing is, like, the thing that sort of led to this, so yeah, I was working in some model system before. And we have another iteration that we've gone through has gone from being like non model systems to sort of organism agnostic to say, like, look, we want to be able to study anything here, what if their biology is appropriate, right? So we sort of want to take down the walls to the things that sort of been us and require us to really work on in places that are not as efficient for mining the biology, right? But the funny thing was, like, you know, in my own work, everything that in sort of my academic life, we were geared towards was studying ways in which the organism I was studying was like most similar to humans, right? Like what's really conserved and what gives us the ability and like easier ways to study it more efficiently. And, but I kept landing on interesting biology that was totally distinct from humans, but completely challenged our assumptions about how the biology works. Now, if you if you don't really understand the fundamentals of how the biology works, like, it doesn't matter how close do you get to humans, right? Like your your basic assumptions are wrong, right? And so, you know, I began to get more and more interested in the ways that biology was unique in these different organisms. And then if we could find out the ways in which they were unique and changed our understanding of the rules of life, and ways in which we could deploy those things to make and engineer solutions for humans better, even if that's not something that exists in humans, like that seemed to me like to be a very useful thing. And so I will say that now we're interested in doing a lot of these things, which is to say, can we mine from a very broad range of biology, but can we also figure out where to deploy it in, you know, a set of organisms that might be more tractable, but really upending our assumptions about that and what what those assumptions need to be. So yeah, so I will say that, you know, originally, we started in very practical terms, right? We didn't really have a lab at first. And then we were in an incubator space. And, you know, we didn't have a vivarium. And so you can imagine that like, constrains the types of things that you can work on. So what we went for originally were protists. And the reason for that is that there's, you know, a huge number of huge amount of diversity and protists across a huge number of clades that you get representatives that are technically protists, you know, and they're single cell organisms. So they're, you know, often easy to work with, easy to grow. In many cases, you could sort of order them from different culture collections and things like that. So we sort of did the simplest possible thing that had sort of like a low regulatory lift, huge amount of diversity. So that's one of the reasons why we started, you know, working with protists. And there were some really interesting things like amoeboid algae and things that look like they combine characteristics of different organisms you didn't think could exist in one, you know, like, it's just, there's a lot of really interesting things that come up in this way. So I will say a lot of our focus has been been and these sort of simple systems, but this, like this arachnoid algae is one of the most interesting is sort of like behaves very much like it looks like it's got nanotubes and other, you know, sort of structures that look very much like human cells and then it otherwise behaves like an amoeba. It's just a very, very interesting set of organisms. And like some of the biology that we've seen around here is like, is quite wild, but yeah, all sorts of symbionts and other types of things where, yeah, I think there's no shortage of awe in different types of things that we would have in-house. But again, I would say that another big shift that's happened in-house is that we sort of started out thinking like, oh, we're going to work on XYZ thing and we're going to land in certain places and whatever. But I think because we're really focused on thinking about how to mine biology more efficiently, there is no way to do that at this point without a computational approach first, right? If we really wanted to try and figure this out, it's going to be really again, mining genomes and other information doing, you know, structural prediction and protein structural search, things like this. Like there's just going to be for us to sort of expand that search space and refine it. It's going to require some computational approaches to sort of mine a broader range of biology than what we could ever do if we put hand to glove first, right? And so I would say that has been one fundamental surprise and shift is that we're doing things like mining biology from marsupials to amoeba and everything in between. But then when it comes to, you know, is it worth us actually bringing things in-house, getting them up and running, trying to make a really sort of bespoke solution to trying to get something to work in house? That bar is pretty high for us at this point. Very cool. I'm wondering if you can elaborate a bit more on the science publishing side of things. So you, as we introduced already, you've been very active in this world. So we talked about ASAPBio, said sort of it's a non-profit organization, promotes transparency, innovation, science communication. But you were also previously on the board of directors at eLife, who sort of consistently innovated in their publishing model. So I'm curious why you think we need to reform the science publishing system and a little bit about how you're going about this through ASAPBio and what you're doing at Arcadia. Yeah, sure. So yeah, I would say, okay, so why do we need to actually reform this? Because I think that in many ways, science publishing is not fit for purpose, right? If we say that the goal for sharing our science is to get it out into the world so that other scientists can use and build upon the work, then we should do that. And I think that what we have now is a system that is largely divorced from that goal. I know it feels like it because we're all used to it, and that's the only thing we've ever done for the most part. But it really is something where one major problem is that authors aren't really, it's not really in their hands when they share the work. The vast majority of the traditional system is pre-publication review, the idea that we need to protect all of the universe from science unless it's been vetted by two or three secret people, which I think is preposterous. I just honestly think that that is putting that much power in the hands of a few people that are the only ones interested to share that information is a terrible idea. And not only for the reason that it's gate kept by so very few, but it's also a terrible idea because the truth is the rigor and quality of science is not determined by what those few people think when they read it at the beginning of its lifetime. What will actually determine whether the quality of science is good is whether or not it withstands the test of time, whether others use and build upon it, whether it's tested orthogonally, whether or not it's been debunked by something contradictory. Testing those models over and over again over time is the thing that will tell us whether or not it's rigorous and whether or not it's high quality. What people think when they read it is just the least possible signal that we could take. And then to say, not only will we use this signal to determine what's good, but we will have all of science dependent on it. Things will not even see the light of day unless this very suboptimal thing happens to me is insane. I really don't understand how we ever landed on such a thing. I do not understand how we got there, but in many ways, the fact that we spend months to years chasing after those three people that tick the box is not only a bad idea, but also fundamentally unsustainable. The scientific literature is expanding exponentially. We are generating more and more and more data faster and faster and faster. If we think that we're going to ask a handful of people who are anointed to just give their time to reading even bigger and bigger and bigger stories that are more and more interdisciplinary, that they don't even have the expertise to weigh in on necessarily, except for one corner of it. It's just not a sustainable solution. In my opinion, it's already dead. People just don't know it yet. We have to now just catch up to the reality of that fact and say, not only is it unsustainable and poorly fit for purpose, but we don't have to do it this way. This version of peer review is not that old. It's in our hands to do something different, and preprints have really revolutionized everything. It's one of the reasons I'm really involved in many of those organizations, because for me, it changed everything the way I thought about science entirely, that really putting that back in the hands, the decision to have things see the light of day back in the hands of the experts, which are the authors. Having that be out in the open, and yes, the thing that everyone's worried about is quality control. How will we know if it's good? The truth is, I can have three people stamp it, you still won't know if it's good. That's what journals are about. I think you've all been torn apart some paper that appeared in some journal, and the truth is, everything that's been rejected from somewhere gets published somewhere else, often as is. That system gives us the sense of security of truth and rigor, but not the substance of it. The question is, are we willing to have our entire scientific enterprise be completely at the mercy of this type of system? I think the answer should be no. I think that there are other innovations, there are a lot of people working in this space trying to say, how can we actually leverage having authors in the driver's seat to share the science, but then figuring out how on top of that can we give some confidence? I think there should be public discussion about science with people weighing in on that very publicly, because that benefits also the readers who aren't sure what to believe, if they don't know whether or not there's been some robust discussion around it. Of course, I believe in peer review in the fundamental definition of it, in that, yes, science gets better when we talk to other people. In fact, the more other people we talk to and finding those experts wherever they live, not whether or not they're in our bin of secret people that we've decided to annoy, but can we actually find those experts wherever they are and actually figure out, have them weigh in on things, but then also even recognize that even with that public discussion, it is really time that we'll determine that. I think we need to get back to a place where the substance of the science is our North Star and not whether or not we're racking up the lists and the accolades and the numbers to make that happen. I think too much have we really been at the mercy of those who are involved in evaluating science to dictate how it progresses, because they will always tell you, but then how will I know if it's good? The truth is, making you feel like it's good when we can't know that isn't really doing anyone any favors. That's generally why I think we need rethink on this and it's really held back science. It keeps things going much slower than they have to go. It's one of the reasons that Arcadia, we said we're never going to publish in journals because we don't have an interest in really slowing our science that way. It's one of the reasons we also don't do traditional academic collaborations because we're not going to put our resources and energy and effort towards working with someone and then to say, oh yeah, but why don't you keep it secret for a couple of years for your nature paper? Not going to do it. It's never going to happen. It's more important to us that we get it out into the world and that people start to use it and build on it. That is the ultimate test. The usefulness of the science is what will really tell us. That's what I always say. I don't know where Alpha Fold is published. I just know that it's changed everything and we use it for everything. I don't need to know. Using it is the knowledge. I think that that is a bar that we should try and meet is to try and say, can we actually do the kind of science that impacts other people, that impacts other science? Our publishing models need to really be geared towards figuring out how can we tell if that has happened? How can we give confidence to other types of questions, which is what kind of science should we build policy on? Maybe that should be the type of science for which there has been rigorous testing and reuse. You can say things like COVID-19 preprints. This is something where unequivocally scientists sharing directly their work as preprints had an unambiguous impact on us getting to vaccines sooner. It's something where it was really urgent, but by virtue of everyone working on the same problem and really rigorously testing each other, it resulted in not less rigorous science. It just happened faster and better. I think these are the types of things that yes, does that scale with every single corner of science? No, but neither does our current system. Let's not sacrifice everything for this obviously flawed situation. Great. I think building on enabling public discussion about science or helping to build community and pull back that curtain, or not pull back that curtain, but open that curtain that might have been a little more difficult to pull back in the past. Earlier in your career, you founded the new PI Slack channel, which is a place for new PIs to share ideas, exchange information, support one another. I see it all over social media. I've been a part of it for a couple of years. It's really been useful in getting alternative perspectives on what it looks like to be an investigator and do science. What did you learn by doing this? Are there other ways you think that we can support early career researchers in this new era and age of science publishing and science in general? Yeah, absolutely. I learned a lot from that. I think I learned a lot about experimentation. I think one of the real goals of that effort was to say, look, everybody's in the same boat who's starting a lab. There's similar challenges. Everyone's going to have the same problems, and they're going to try and find ways to solve them. The way they do it now is to have some mentoring committee or whatever, if they even do. The truth is, we have a vast amount of data, which is every single other person going through it at the same time. Everyone has their own experience, and not only are their own laboratory, but their own laboratory of experimentation for how to run a lab. We have a lot of data out there. Are we leveraging that data? Now, I think we are in biology learning how to actually use machine learning to leverage all of the data that exists in the universe to do important and interesting predictive biology. In that very nascent part of my career, I really thought, well, why can't we leverage all of the information that's out there from other people going through similar challenges and learn from that, and in many ways, just crowdsource the solution? The idea wouldn't just be to have a consensus solution. It's not to say, oh, let me take a vote of 3,000 people and decide what to do. That's not the goal. The goal is to say, let me at least hear about the experiences of other people who have tried things that I haven't yet tried or who have done things I have not yet done, and have that inform my decision. To say, with all of that data in hand, now I have a new opinion. The thing that really influenced me was to think about experimentation. The ways you can see that manifest itself now is that's how I think about Arcadia and what we're doing here is it's an experiment for how to run an organization. I often say we don't claim to know the right answer. We just know it's important to try and important to iterate on. This is something I would really encourage all early career researchers to A, just have the agency to say, look, you can be that. You can be your laboratory of experimentation for anything in your career, how you decide to run your experiment, to deal with your mentor, to collaborate with others outside, to decide what you care about in publishing your own work. All of these things, I think that there's so much conventional wisdom out there on how you should follow what rule book to progress in your career. I will be honest that I don't believe any of it. I think the reason why is that I think that all of us are different. We all have unique strengths. I also don't think that the very best way to really leverage your own strengths is to try to be just like everybody else and become a crappier version of yourself. That is not a path to any kind of success that I know of, even though that's the thing, that everyone is racing to run the same race instead of running their own. I don't really understand that. I think that it's really, really important to really think about taking an input, making your own decisions, and figuring out what is the way in which you are going to run an experiment for how you think you should navigate science. Anything is on the table. I know that it doesn't feel that way to a lot of people and that it feels like they're constrained in all of these ways and there's a set of rules. I will say that there is more optionality than you think. It's one of the things I actually loved about academic life is that you could really do science in whatever way you wanted. In many cases, it doesn't feel like it. It feels like this is what you have to do, that these are the incentives. It's a word I hate more than any word in English language because I don't believe that you have to do what's best for you to do what's best for science. I think that there are many more degrees of freedom and the more you can figure out how to leverage your strengths to do the types of things that you're uniquely good at and can bring something really impactful to the world, if you make an impact, people will know it. It's not saying, oh, just put your head down and do science because I don't think that's not what I'm saying. I'm saying that there are definite ways in which you should think about how it can be better. In many cases, there's a lot of room for improvement. To me, it feels like we all have that in us and I hope that early career people feel empowered and can feel like they can support each other because everyone thinks something different. Everyone will tell you something different and it's important what you do and what you bring. You've got this one life, what are you going to do with it? I really think that it would be great if young people supported each other. I think that's how change happens. I don't think that we can say we expect everything to change if none of us are willing to change it. Yeah. We're starting to come towards the end here and I think building off of that, you've touched on a couple of things in your last answer about support one another, don't just blindly follow incentive systems, but I'm wondering if you have any advice for researchers who are interested in testing out building new models of science, testing new models of entrepreneurship? Well, first of all, I'm very excited. I think that this is unprecedented times for science, unprecedented times for biology, and I think there is no better time in the present to really try and explore those things. I think really stress test your ideas, talk to people about them, widen your networks, the things that are true that you already know how to do in your academic life. You already know how to network, you already know how to meet people, but the truth is that's still a very small corner of the universe. Get out there and talk to other people and learn some things about where is the interest in the type of thing that you're trying to do? What is it that is your competitive advantage and what is it that you think is truly unique? You should always ask yourself that. What are you doing that could move the needle? What could that be? What should that be? I think that you'd be surprised at the degree to which people are willing to and excited to help and contribute to that and share their feedback and their ideas. Because I think that there's just an enormous universe of people out there that just want to innovate and do better things and want to put resources towards better things. And you're looking for them, they're looking for you. Just get out there and really try and hone your ideas and hone your message and really spread your networks to try and identify what are those different paths. And I can't say forcefully enough, getting out from your own bubble and your own silo and whether it's social media or any other networks that you have, getting out there and outside even where you think your comfort zone is, I think that's where the real gains are to be made. Because I think all of us feel comfortable in some areas. It's really exceptionally exciting times. So I'm excited for you all. Yeah, well, I'm very excited and motivated now. Yeah. Just with the last couple of minutes, just wanted to give you the space and opportunity. If there was anything that you wanted to talk about that maybe we didn't cover or if there are any initiatives, projects or events that you'd like to highlight or promote to the audience. Yeah. So first I do want to say that our science is going out on our website, but the other thing that we're sharing is our sort of meta-science experiment. We're trying to sort of build in public as well so that everyone who does want to start a new organization can learn from our lessons. So one of the early things that we did was really go and talk to other people who had started organizations and try to figure out if they could do it again, what would they do differently and all of these other things. And everyone gave us this universal advice that, oh, just make sure to write stuff down because you'll forget all this stuff and you're head down, you're so busy building something that you never tell anyone. And it's universally true for everyone because no one did it because everyone's got their head down trying to build an organization. And so they never share that stuff. And sure enough, we made the same mistake. Everyone said, oh yeah, make sure you document X, Y, Z thing. And even us, two years in, we still have not done that to the degree that we would have liked, but we really are committed to doing this because we want, again, the impact of this organization existing to influence and help others who might think about iterating on what we've done, even if it's trying to avoid things that we failed at, whatever it is. That type of information is also going out on our website. So I strongly encourage you all to engage with us, to make public comments on our pubs, on any of them, on how we're building this company, but as well as the science itself. And we're doing more to get out into the community to think about people using the things that we're building. Of course, we're always recruiting. So you can check the careers site on our page. We are still at about a third of what we ultimately think we might grow to. So we're going to continue to recruit in the coming years. And so always looking for people who are interested in building something with us that is, yeah, might not exist today. So our website really has all the ways to get ahold of us. So very excited for you all to engage with us. If anything, the one thing I hope everyone does is feel excited and empowered to go and do something. I think there are a lot of people who talk about things and don't do them, and it can feel like a scary thing. Not everyone can. There's constraints and all sorts of things. But to the degree that you are able to take liberties and make things happen, I very much think that there's always things that we can do. And that is really our path to a brighter future for science. And I don't think that anyone needs to give you permission to do that. It's in your hands and I can't wait to see what you all do. Wonderful. So much to be thankful for there and really excited. And yeah, just thank you so much for coming on the podcast. It was really awesome chatting with you today. Yeah, thanks so much for taking the time. Thanks for asking me to do this. It was really fun. I was really nice to meet you both and I'm sure we'll cross paths again. This has been another episode of EBRC in Translation, a production of the Engineering Biology Research Consortium Student and Postdoc Association. For more information about EBRC, visit our website at ebrc.org. If you're a student or postdoc and you're interested in getting involved with the Student and Postdoc Association, you can find our membership application linked in the episode description. A big thanks to the entire EBRC SPA podcast team, Andrew Hunt, Ross Jones, David Mai, Heidi Kumpa, Rana Saeed, Will Grooby, Matt Williams, Ice, Chonfisit, Kiatasui. And thank you to EBRC for their support and of course to you, our listeners, for tuning in. We look forward to sharing our next episode with you soon. Thank you.