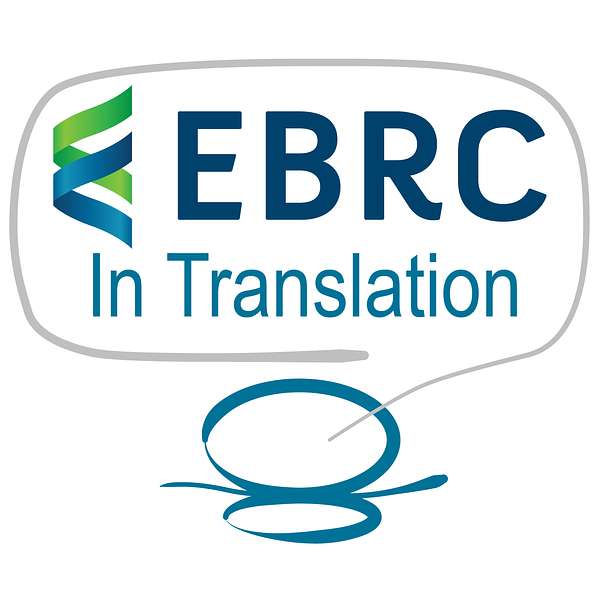
EBRC In Translation
EBRC In Translation
20. Control Systems for Gene Expression and Work-Life Balance w/ Mustafa Khammash
In this episode, we are joined by Dr. Mustafa Khammash, Professor of Control Theory and Systems Biology in the Department of Biosystems Science and Engineering at ETH-Zurich. We talk with Mustafa about integrating control theory into synthetic biology, designing computer/biology interfaces, starting a wet lab as a tenured computational professor, the need for new theoretical frameworks for biological design, and more!
Notes:
During the episode, Dr. Khammash references two different papers from his lab, linked below.
Cybergenetics: Theory and Applications of Genetic Control Systems
Universal structural requirements for maximal robust perfect adaptation in biomolecular networks
For more information about EBRC, visit our website at ebrc.org. If you are interested in getting involved with the EBRC Student and Postdoc Association, fill out a membership application for graduate students and postdocs or for undergraduates and join today!
Episode transcripts are the unedited output from Whisper and likely contain errors.
Hello, and welcome back to EBRC in Translation. We're a group of graduate students and postdocs working to bring you conversations with members of the engineering biology community. I'm Ross Jones, a postdoc in Peter Zanstra's lab at the University of British Columbia in Vancouver, Canada. And I am Reina Saeed, a PhD candidate in Nair's lab at Tufts University in Medford, Massachusetts. Today we are joined by Mustafa Kamash, Professor of Control Theory and Systems Biology in the Department of Bi-Systems, Science and Engineering at EDH Zurich. Thank you so much for joining us today. Yeah, it's my great pleasure to be here. Thank you for inviting me. To start off, can you tell us about your journey to becoming a professor and what drew you to control theory and synthetic biology in particular? Thanks for the question. Even though I'm in EDH Zurich right now, I spent basically my whole adult life in the US, including all of my undergraduate and graduate education. So I did my bachelor's at Texas A&M University in electrical engineering. From there, I went on to Rice University in Houston to get my PhD in control theory. While at Rice, I got exposed to the idea of control systems. And I like math a lot. And within engineering, control theory was particularly mathematical. And what was particularly appealing to me about the field is that control systems are present everywhere. So they're in electrical engineering devices, mechanical engineering devices, chemical engineering devices, and so on, aerospace systems, biology, ecology, and so on. Yet, by abstracting the control systems in all of these desperate fields, one can arrive at a mathematical formulation where you can make contributions. And then the contributions that you make can be applicable to all of these fields. This seems particularly appealing to me. Once I've started doing research, I was hooked. And so I was drawn to control theory early on. And I think this has been mostly the one field that has defined my career since those early days at Rice University. Different applications. I've worked on power systems. I worked on aircraft control systems. I've developed theory, robust control. And then over the last, I would say, 20 years, I've been working on systems and synthetic biology, perhaps almost exclusively now. And in terms of synthetic biology, the work that I'm doing now is, by and large, mostly synthetic biology. And the reason why I was drawn to synthetic biology has more to do with the nature of engineering and control engineering. So control engineering involves a lot of synthesis. So you build systems. And one of the key questions is, how do you design or how do you synthesize a control system, whether it be for an airplane or a car or any other device? And after I was drawn to biology, this was in the, let's say, late 1990s, early 2000s. Much of the work that I've done had to do with analysis and more like systems biology, more reverse engineering. And I think this was interesting. And one can still use the tools of system theory and control theory to get a deeper understanding of biological complexity. We had a lot of fun in those days trying to understand biological systems. But then when I joined ETH and started my own lab, this presented an opportunity to start doing forward engineering or to design biological systems. And synthetic biology is exactly doing that. And so I jumped at the opportunity. And since 2011, I never looked back. Maybe I want to go back a little bit and tell you how I got interested in biology in the first place. I mean, that's another interesting story. In the late 1990s, I was at Iowa State at the time, I was a professor at Iowa State University in Ames, Iowa. And my wife had an illness after giving birth to our first child. Something to do with thyroid glands, so going through periods of hyperthyroidism and hypothyroidism and so on. I didn't really know much about this area at all. So I went to the library and picked up a textbook on endocrinology and started reading it. And I was really shocked. Essentially, everything I was reading in this book was basically control theory without the equations. One feedback loop after the other, after the other. All exquisite control systems were described in words, not in equations. I was really very intrigued and very interested in going further. At that time, I had a young master's student that just joined my lab, Hannah El-Samad, who's currently a professor at UCSF. She's also recently joined ALTOS Labs, she was a master's student at the time. And so I convinced her to try out biology with me. We went to a professor, we went to professor in the National Animal Disease Center in Iowa. And I basically introduced myself as a control theorist and I was interested in the type of problems that require feedback regulation. And so he gave us a problem that I think was perfect for us to start with. And this is the problem of understanding how mammals regulate calcium in their plasma. It was really a wonderful research question to start with. It was simple enough, yet there was a lot of uncertainty and it was also related to a particular disease. At that time, they were studying dairy cows and the disease was called milk fever. So it had all of the elements, all of the aspects of a perfect research problem to work on. We started by looking at the way that mammals regulate calcium normally, not the disease state, but just the normal regulation. And this led us to really discovering integral feedback control in dairy cows. And this was a very, very exciting discovery for us at the time because even though we didn't really know the details of the endocrinology or the different hormones involved, one can essentially deduce them by applying basic principles, even undergraduate principles from control theory. If the system is adapting perfectly to increase calcium demand, there has to be an integral feedback controller in the loop. The question is, how would you implement an integral feedback control using molecules? And so one could speculate about one molecule, maybe one needs to have two molecules, and then one can then try to theorize about what sort of interactions these molecules would have with each other. And then once we've done that, we went back to the textbooks and we indeed found that there are two hormones that worked exactly the way that we thought they should. One of them is parathyroid hormones called PTH, and the other one is a form of vitamin D, has a long name, 125-dihydroxycholecal-speral. Both hormones have to interact dynamically with each other in a very specific way in order to implement integral feedback that is needed to achieve perfect adaptation in dairy cows. So this was kind of like a very early success, and it was enough of a success to kind of attract us both to the field of biology and move on to the next level, which was studying things at the molecular scale. So this is how I got started. From then on, I went to Caltech to visit John Doyle for a faculty leave on a sabbatical. And working with John, we worked on this problem of heat shock response in E. coli, very, very different from the calcium homeostasis problem. But that's also another, I think, milestone for me, because that is where we first realized the need to look at stochasticity and stochastic models in biology. And the story there is very interesting. So we were modeling the heat shock response using data that was given to us by one of the world's leading experts in the field, Carol Gross, who was actually still a professor at UCSF. And one of the things that struck us when we developed these deterministic models is when you look at the concentrations of some of the key molecules, the sigma factors, the model would indicate that the concentration is about what would be equivalent to about 0.1 molecules per cell. OK, this seemed completely absurd. What does it mean to have 0.1 molecules per cell? So this kind of forced me and Hannah and John to kind of look at different ways of modeling these systems. And at the time, there was this work by Adam Arkin on the lambda switch. And we have just introduced to Dan Gillespie, who was at China Lake at the time. And it seemed like looking at stochastic models was the perfect thing to do. We didn't get into this willingly. Actually, I got into the stochastic analysis kicking and screaming because my tools preparation didn't prepare me really for this type of model. Most of the work I had done until that point was deterministic dynamics. But there was no escaping stochasticity. So we had to learn this new math and this new area. I think that has been really a key moment for me personally, because much of the research work that I've done since then actually involves stochastic systems. So anyway, that's in a nutshell how I got into systems biology and from then on to synthetic biology. Awesome. That's super interesting, especially to hear about the different sort of introductions that you had to the biology side. The first thing you touched on was moving to ETH and sort of adapting your lab at that point. I'm curious how it was coming from a theory background, building that lab side of your lab, which has now expanded from working in bacteria to working in mammalian cells. So running quite the gamut there. What was your approach to making that transition? When I moved to ETH, I didn't even think about starting a lab. But during my interview, I was asked if I was interested in having a wet lab. It wasn't something I'd thought about, actually. I think I just quickly said yes, thinking that, well, if I am to have a lab, this is the time to do it when you're negotiating with the president. And if it didn't work out, I just closed shop and continued doing what I'm good at, what I've been doing all along. At that time, I had the impression that maybe they will give me one or two benches where I can actually do experiments on the side just to verify one concept or another. Then when I was introduced to my lab, it was this massive lab with eight benches and all kinds of equipment. That was kind of a terrifying experience. And so I never thought I would fill it. I would just still use maybe a corner of it. My approach was to essentially my first hire, actually somebody who came with me from University of California, Santa Barbara, where I work, is a molecular biologist. I was very fortunate that at the time, postdoc Stephanie Aoki, she was considering to move to Switzerland with her Swiss boyfriend, but didn't really know where to go. And her advisor told me, look, if you accept this offer, I have the perfect person for you. So I accepted, you know, Steph came along and she was a molecular biologist, so she helped me get the lab started. And I think that that was key, you know, to have a real experimentalist to start the lab. Once Steph came in, she was followed by other experimentalists. I think then for me, the next move was to try to integrate the engineering aspects and the theory aspects into the experiments. By looking at an approach where experiments and theory work together hand in hand, and I think this has been, you know, something I've worked at since those early days. And I think this has worked quite successfully, and to the point where now the theory drives the type of experiments are done, and also the type of data that can be measured experimentally, they define what sort of theoretical questions models should be developed and what questions should be asked. So now both theory and experiments work very closely together. So we have a rational approach to experiments. Now everybody in the lab, for example, is experienced in building models. I tell my students and post-docs never to build any circuit that they haven't first verified using the mathematical model, and that it works, and it works reliably, not just for a very small range of parameters. If it doesn't work in a computer model, then there's no point in spending the time and building it experimentally. So this sort of thinking of combining, you know, modeling theory and experiments together very tightly, I think has been a key to success. The people I hire, they come in different varieties. So some people are molecular biologists, they're interested in doing experiments primarily and being exposed to models all the way to pure theoreticians and everything in between. Some people actually would like to do both and do do both. So they develop their models and then they go to the lab and build them. And I'm somewhere in between trying to kind of bring the two ends together. I'm impressed about the transition into biology and the experiment of having these two hand in hand to solve a sensory biology problem. Your work has emphasized the stochastic analysis of genetic suppression, an approach that is all too often ignored in molecular modeling. What have been your most important high-level insight from analyzing stochastic behavior of genetic circuits, especially when it comes to feedback and feedforward control? Yeah, thank you for the question. I mean, motivated by our early experiences in modeling the heat shock response, one of the things we have found also in the lab while doing synthetic biology is that there are three different regimes to look at, three different modes of representing biological systems. The first one where we would like to be working in, which is deterministic, of course, you build deterministic models. These are either ordinary differential equations or partial differential equations, but they're still deterministic. So that's one mode. The other mode, you look at actually modeling the population, right, population-level models, looking at population averages. And the third one is looking at single cell models. And one of the things that one finds out early on is that these can have drastically different behaviors. So what your deterministic model tells you may be quite different from what even the average population-level behavior looks like, which would in turn be different from what the single cell tells you. And of course, the tools and the levels of complexities of dealing with these three modalities are actually quite different. So one has no choice but to embrace all of these three and be able to switch back and forth. Now, what, and of course, the reason why one has to worry about all of these is because of stochasticity, because, you know, at the molecular scale, the noise gets amplified and one can not use the, let's say, law of large numbers to arrive at ordinary differential equations generally. And one has to live with the fact that the cellular environment is noisy and stochasticity is part of life there. What that means is that one needs to look at new tools, very often more challenging and difficult tools and less developed tools, but also the conclusions one draws about cells could be more realistic. Looking at population level, for example, because of stochasticity, systems may be stable, whereas looking at the same systems deterministically without the noise, it could be unstable. We've seen examples of that. And so if you want to know what actually is going on in the cell, you have to be able to go back and forth between these different methods. One of the things that we have found out is that, you know, if you look at what's going on in population average, of course, it's different from the single cell and variability cannot be captured by deterministic models. One has to look at stochastic models, but also several interesting phenomena are the byproduct of interactions of the stochasticity with nonlinear dynamics. And so this makes the field much richer, although also, as I said before, more challenging. When you're building controllers, when you're in the business of building control systems, you can find topologies that give you, let's say, integral feedback controllers that work perfectly well in the deterministic regime. But when you introduce noise to them, they would fail spectacularly. On the other hand, you look at other topologies that are much more robust to the stochasticity or to noise. And to be able to discriminate and distinguish between these different topologies is important if you want to build these systems and if you want to have them work reliably in a cell. And so I think that really there is no escaping stochasticity if one is in the business of building circuits at the molecular scale. I wouldn't say this is a negative. I think, you know, as I said before, there's an added richness in dynamic behavior that is introduced by stochasticity. So it makes math problems more interesting, and I think it also makes behavior and functionality also more interesting. One of the benefits, for example, of using, let's say, adopting kind of a stochastic approach is that these methods tell us, for example, that one is able to build control systems that using a very, very small number of molecules, maybe one or a handful of molecules, and that the systems should work, okay. Why is this important? Well, I think a lot of the challenges with synthetic biology have to do with burden and being, you know, using a lot of cellular, a lot of the resources of the whole cell. So if there's a way to build genetic circuits that have a very small footprint, that don't use up a lot of molecules and still have them work reliably, then this should have a clear advantage. And that's what we actually see. That's what we see when we simulate these systems, that's what we see when we actually build them. And so there is benefit to also the stochasticity, it's not just a nuisance, and I think, of course, cells have evolved to exploit stochasticity in a myriad of ways, and I think there are a lot of reports on how our cells benefit from this noise, but I think so, too, can synthetic biologists who are interested in building reliable circuits that have, let's say, use up as few resources as possible. Okay, that was really interesting, Mustafa. Thanks for your perspective on that. When you were telling us about your background, you introduced some ideas where you had been looking at control theory problems that exist in biology, but some of the work that you've been doing more recently is sort of forward engineering control systems within cells. And I was wondering if you could quickly tell us a little bit about the problems that you see this being useful for solving in the engineering of cells. Why should we be using feedforward and feedback control? I think if I were to answer this in one word, it's robustness. And in fact, let's say in the particular case of feedback, but also feedforward, there is really no advantage to feedback control if the systems that are being controlled are not uncertain and not variable. And so biology has both, it has a lot of variability and a lot of uncertainty, and even more so than the typical engineering system. And so if one is to design functionality in a reliable way, in spite of this uncertainty, in spite of the perturbations that could affect the system, then I think there's no escape using these sort of strategies. And so both feedforward and feedback control endow the system with robustness. Now of course, there are differences between feedforward, let's say incoherent feedforward and feedback that are important. So for example, in the case of incoherent feedforward, usually these systems tend to be simpler to design and build, but they're robust usually to a single input that you know in advance. On the other hand, feedback control systems tend to be more complicated and more difficult to build, but then they can give you a robustness to a larger class of uncertainty or let's say a variety of different perturbations. And they could also lead to dynamical systems that are unstable if one is not careful in the design. And so, but the bottom line is that both types of control strategies allow you to build systems that deliver functionality in the presence of uncertainty in a reliable way. So you have been developing the cybergenetic tools that work both within the cell and without using computer interfaces. What do you see as the strengths and weakness of each approach in the most exciting applications? Yeah, I think so as you said, we have had two different approaches to building control systems. One in the first approach, which is probably less familiar to your listeners, is an approach whereby one interfaces living cells with computers through various types of interfaces. For example, light or let's say chemical induction, which is delivered through microfluidics. And so the signals, the control signals are instructed by a computer that's simultaneously reading out the behavior of the cell through, let's say for example, fluorescence. And so one can devise feedback control systems where, you know, these hybrid systems where, you know, part machine, part living cells. And you can do this, we've developed technologies to allow us to do this both at the population level but also at the single cell level where we can control a large number of single cells independently with each of those cells under the microscope, each of those cells having its own independent computer controller that is running in parallel. Now this approach has several advantages. One of the advantages, of course, you can build very sophisticated control systems inside the computer and have them control real living cells very quickly. If you don't like the behavior, you can change a parameter, hit return, and then you can try out the new parameter. And so this allows you to do rapid prototyping. So if you would like to build a new genetic circuit before you actually go ahead and spend weeks or months to build it by using genetic techniques, you can put it in this, what we call the cyber loop, where that circuit is running in simulation to control the real system. And then you can explore its behavior in this environment. And by varying different parameters or different topologies, you can even introduce noise, you can adjust the level of noise and so on. And only when you're happy with the behavior of the closed loop system do you start building it using genetic methods. So this, I would say, rapid prototyping is one clear advantage. Another advantage, for example, you can apply, as I said, you can have very sophisticated control systems that are virtually impossible to build using genetic methods. You can have advanced model predictive controllers, multivariable control systems running, let's say, controlling a bioreactor with light using optogenetics. You could also use this approach to study cell-to-cell communication. So by reading out the behavior of a multitude of cells and being able to control the expression of each one of them independently with light, something we can do using a digital micro devices, we can simulate the communication protocols between different cells and see how different communication protocols impact the behavior or, let's say, the pattern that develops between these cells. So all of these are actually nice advantages of the sort of outside of the cell type of control. But of course, there are clear limitations. So for example, if you would like your cells to be autonomous, then you have to build a controller in an embedded way. It has to be genetically incorporated inside the cell. And a very good example of that is, let's say, cell therapy. In a cell therapy application, if your cells, genetically engineered cells, T cells or whatever, are to be circulating in the blood, there's no way to be chasing these around with light. So the only way to do it is to implement the controller inside the cell. There are large bioreactors where the density of the cells is so thick, it's impossible to deliver light inside the cells. Again, you have to genetically engineer these things in there. And other examples, so if you want to have, right now we can only do single input, single output control with the computer, mostly due to the limitations of the present day limitations of optogenetics. But one can imagine that you can implement a multitude of, let's say, multivariable controllers inside the cell, maybe with access to different molecules that may not be easy to measure externally. So again, I think these are complementary methods, and we work with both, and I think each has its own advantages and disadvantages. Of course, the genetically engineered cells, they take much longer and much more difficult to design, engineer and build and have them work. But for these type of applications that I mentioned, I think there's no way around it. It's really cool thinking about using it as a prototyping system for a circuit that you could put inside of a cell, I hadn't really thought of it from that perspective as well. And I think that leads in nicely to the next question about how biologists and engineers sort of approach problems differently. So as somebody from more of a theory engineering background, what do you think that biologists that are sort of wanting to get into the space, what modes of thinking or concepts do you think would be helpful for them to dive into the theory and computational side of the work, and maybe vice versa for somebody coming from a more engineering or computational or theory background, what biological concepts do you think are the most important for them to be able to understand? Yeah, right. So actually, for biologists trying to get into computation and theory, I would say from my experience working with students with a pure biology background, I think there are two things. One is developing, I would say, a dynamical systems view of biology. OK, so this need not be time consuming. I mean, maybe a very simple course or just reading a textbook like that of stroke ads, for example, on dynamical systems would be sufficient. But I think it is really extremely important to be able to understand, have a dynamical view of biology, generally of the world, but in particular, if you're working in biology of biological systems, you know, simple concepts like fixed points, stability, steady state behavior, oscillations, bifurcations, these type of notions, they're not difficult. But I think to be able to understand them qualitatively, I think is extremely important to kind of make sense of biology. And as I said, they're not particularly difficult to learn, but they're super important. So this is one way of thinking about biology in terms of these type of concepts. Another thing is something we've mentioned earlier is understanding the key role of variability in biology. The average behavior is not really doesn't really represent everything that we look at single cells, they could be doing very different things and understanding that, appreciating it and being able to to model it, I think would be key. Now, for engineers and theoreticians in general, you know, engineers, physicists, you know, quantitative getting into biology, I would say, I mean, that included me when I got started. I would say one of the few things I would say being able to understand what can and cannot be easily measured in biology and what type of data one can expect. That is usually something that is very underappreciated. Perhaps, you know, we're spoiled as engineers, you know, if you're building a radio or working with an electric circuit, you don't really understand or see why you can't measure this variable. You know, you have an oscilloscope, you want to measure a voltage across this resistor, you know, you just, you know, put your oscilloscope there and you see the voltage, you know. So you have an entire view of the inner workings of your circuit. Wouldn't it be nice to have a biological oscilloscope where you can actually measure all of these intermediate variables in real time? And that's something that is very difficult to have, but it's something that's really underappreciated by newcomers into the field with an engineering background that, you know, there are very limited things that you can measure and even those that you can measure, the type of data that you can expect is also rather limited. As a result of that, to be able to accept that biology has a large amount of uncertainty, both in the in terms of the the players that are involved in a particular network, but also in the interactions among these players. So this is really very underappreciated. Usually when you're working with a mechanical system, electrical system, you know, you pretty much understand all the different parts and how they're interacting, but not so in biology. Right. I mean, that's just the fact. And so as a result, I think there needs to be one of the things that I think newcomers or computational engineering need to be able to respect the sheer complexity of biology and not really to compare it to engineering. I think that, you know, everybody who comes into this field has to come to grips with those three things. Yeah, it's a great advice for biologists and engineers as well. And I got a lot from the points you mentioned coming from molecular biology and biochemistry and then doing PhD in biological engineering. So I understand what you're saying. My question now is like most of the SPA membership is in the US. And but many of us are really interested in knowing what this is like in Europe since you have led both a lab in the US and in Europe, what can you tell us about the differences in the environment or in the funding allocated research, like how PhDs and postdocs do their training or any other aspects of the research? Yeah, I think that's a very good question and something also I had to get used to, you know, switching to a new system in Europe. I mean, generally, you can start doing research, start working on your PhD coming out of the bachelor's degree in the US. In fact, that's what I've done. I never really got a master's degree. I just it was a master's PhD program. I got I went straight from the PhD and a lot of US programs do that. Not so in Europe. I think in Europe, you really have to have a master's degree. And it's part of what's called the Bologna Convention 3 plus 2. So three years of bachelor's plus two years of master's or in combination, they're like bachelor's bachelor's and sorry, sorry, the bachelor's 3 plus 2. And then you start entering into the PhD program. You already have a master's degree. And as a result, you know, entering PhD students tend to be older and more experienced in Europe. So this is one big difference in terms of work style and work environment. I would say the US students and postdocs are overworked. Maybe it's not a surprise to you. I see you're nodding. So I think that there seems to be maybe peer pressure or I don't really know where it started or maybe it's just it's not just at universities. I think it's you know, the average job also in the US as can be similarly characterized. I think there's a peer pressure to, let's say, work for weekends and after hours and so on. And there's none of that here. In fact, the typical European students enjoys five weeks of vacation and they really use it. The other day, I happened to come to my lab in the weekend because I was in the building at the time. I could only find one person and usually there isn't anybody. And that's just the way it is. I think, you know, there's I think in Europe, there's a better, let's say, life work balance than in the US. I mean, I say that I, you know, as I told you before, I spent mostly all of my most of my adult life in the US. So I'm myself consider myself as a workaholic. So I work weekends and so on. And after hours and I it's not so easy to drop everything and really take advantage of these long holidays. I would say one of the most interesting and amusing things I found when I first moved to Switzerland is a law in Switzerland. I'm probably going to massacre this for the German listeners. It's called Erholungspflicht, which translates literally to vacation duty. So it's your duty to, you know, to take your vacation. And of those five weeks, the duty is that they have two of those weeks have to be consecutive. So, you know, you have to you cannot just chop it up because you're not relaxed enough. So you have to take consecutive vacation. I have to say I'm derelict in my duty. I have been since I came here. Don't tell anybody. But this is I think this is this is something that you see again, not just in universities. I think it's everywhere in Europe. And I rather like this, even though it's taking me a while to kind of kick back and relax and take advantage of this. One would think that this, you know, maybe you will get less done that way. But I have to say my experience has not been like this. I think the students that really stop working and never show up in the weekends and never work weekends, they come Monday morning. They're very fresh. They're, you know, they're very efficient. And it's not clear to me that you get less done. In fact, if anything, maybe you get more done. You know, that's that's I think one of the biggest differences. In terms of research funding, I would say, you know, I'm mostly familiar with the Swiss system in Switzerland. And so I can tell you in Switzerland, it's I think we're in a really nice situation because we have as professors, we get some funding from the institutional fund, which basically makes funding more stable. So there's a lot less pressure to write grants and get external funding. Of course, we all do it. We all get external funding, but there's less pressure. So one of the benefits of such institutional funding is that you can actually do long term risky research without having to worry about, you know, writing one proposal after the other, after the other. And you can work on things that could fail, work on things that could take 10 years before they, you know, yield results. You don't have to worry about writing proposals. So that's, I think, been a tremendous benefit. One of the main attractions for me to, you know, join the ETH. It's not like this in all European universities, I must add. I think this is something particularly, I think, special in Etihad domain, but it's something that I've really learned to appreciate. The other thing is that I think there's less, a lot less defense funding in Europe than there is in the U.S. So the U.S. has a lot of defense funding. And so that's just the way it is. On the other hand, I think for graduating students, there's a lot more tenure track positions in the U.S. than there are in Europe. I think many of the students who graduate, they could maybe go on to be group leaders, which is not really like a tenure track assistant professorship, but a place where they can do some research for a certain duration before moving on to the next level of their career. On the other hand, in the U.S., I think a lot more tenure track positions available. And I would say finally, maybe in terms of comparisons, I think the U.S. still has the best universities in the U.S. are the best in the world. But I think that remains so today, although several other countries are trying to catch up. But I think, you know, if you're fortunate enough to be in those top universities, I think you can be sure that these are the best institutions on the planet. There aren't really that many other differences in a way, day to day life of researchers. But I think these are the differences that I would say stand out the most, at least to me when I first joined. It's really interesting to hear about all that and interesting also to hear your perspective on the work-life balance differences. I've heard that Switzerland is almost militant about having a good work-life balance. And so it's interesting to hear that in practice. So EBRC, our organization, is very interested in creating an inclusive synthetic biology community, both from people from different scientific backgrounds and also personal backgrounds. So we're wondering if you had any insights for the trainees in this field, for us and for the field itself to sort of embrace and enhance diversity, equity and inclusion or DEI. You know, I think that by working with people with different experiences and different backgrounds, one gets new ideas and new perspectives. These are things that wouldn't have been there otherwise. I think this generally enriches the research environment and leads to novel ideas that I think are very difficult to have without this diversity. So I think incorporating diversity in the working environment, again, not just in academia, but basically in every aspect of life, I think is a big plus and is something that we should all embrace. Also, we live in a world full of challenges, and so we need the contribution of each and every single person. And we all win by being inclusive. Aside from just this being basic human decency, I think it is something that has added benefits to everybody involved. And so I think it's up to all of us to make sure that we are inclusive in everything we do and that everybody wins. It's a win-win situation in this regard. And I applaud your organization for adopting this and supporting this. Awesome to hear about DEI and efforts to embrace that. You shared some of the stories behind the track you chose in to be a professor and all that. And I'm sure a listener will be really interested in knowing what is the advice you give for the young scientists thinking about the path you chose and any specific advice for people really want to be a professor? OK, I think this is something I see in my dealings with my own students. Usually about the second or third year, I have a talk with them. I ask them what they're interested in. I mean, they're interested in academic careers or industry, industrial careers. And I get all kinds. And one of the questions is what to choose. Which one of those? I would say it's largely dependent, depends on the person's interests, area of interest. And they're just their own priorities in life. I would say industrial positions are generally better paid, but that academic positions, again, in general, lots of exceptions, come with more flexibility and freedom to pursue the problems of interest. Having said that, I think depending on the area, one can easily pursue state of the art research, also in industry, just the same or even more than in academia. So machine learning is a very good example of this. And so I think it really largely depends on the person. As far as advice for those who want to be professors, I think, well, let me think. I have I can give several thoughts that maybe maybe from my own, these are influenced by my own experiences. One of the one of the pieces of advice that I like is something that actually I've read from an article by Richard Hamming. Richard Hamming was one of the Bell Labs pioneers, and he described his experience in research. I'm going to give you a quote. Hopefully I get it correctly. But one of the striking things that he mentioned is that most scientists spend almost all of their time working on problems that even they admit are neither great or nor are likely to lead to great work. That's very interesting. And then he went on to say, if you don't work on important problems, you're not going to do important things, except by the dumbest of dumb luck. So I think this is, even though it seems obvious, I think this is really profound. And I think that advice that comes with that is that I think it pays to be selective on what problems one works on. So I think giving a lot of thought to what research problems you want to work on is important. I mean, there are a lot of very challenging, maybe even interesting problems to work on that may not be related, may not be important. And I think this was one of the criteria I think that one ought to consider when deciding what to work on. Another piece of advice I would say would be to be constantly reading and learning new things, especially outside of your own comfort zone, your own field of expertise. It may not seem like this is important, but it will pay off. I think after all, I think some of the most interesting and exciting problems these days are at the boundary between disciplines. And if one wants to take part in advancing these problems, then you have to learn something about these other disciplines. So that's, I think, very important, especially nowadays. Another piece of advice I would say that's maybe easier said than done, but I think it's very, very important for a young assistant professor in the field is to learn how to say no. OK. So it may be strange to say this. I think one of the things you find out as you start a new career, there's a lot of demands on your time by all sorts of people and all sorts of sources. And I think that that's just the way it is. You know, it's very tempting to try to say yes to all of them or most of them because they all seem interesting and they are interesting, perhaps, to you. The thing is, your time is limited, and so you have to really, really be very careful about what you do, especially in those years before tenure, but also afterwards. I think if you are to have a good work-life balance, I think you have to be very selective in the sort of things that you work on. And so my advice would be learn how to say no. Not easy, but I think necessary. And finally, and maybe most importantly, you must enjoy your work. And if you don't enjoy it, I think you should just leave it and do something else. Life is too short. Right. That's wonderful advice. I guess moving forward in the future of the Kamash Lab, can you tell us sort of what areas of biology you're getting into and are interested in, and maybe what your perspective is on the future of synthetic biology? Okay. I think these are two different questions. So what sort of things we're interested in? I mean, we're interested in a lot of things, but as I just said, we have to focus. I think that I would like to learn more about the area of development, which includes, you know, stem cell research, but development in general. I mean, nowadays we have new tools are becoming available and we can build fancy things. In my own lab, we're also looking at developing organoids, looking at kidney organoids and so on. And so it's becoming more and more possible every day you read a new organoid type that is being developed in one lab or another. But one of the things that strikes me about this, these discoveries is that, you know, we may be able to build organoids, maybe we'll even be able to make whole organs. But I think our understanding of the underlying development process is lagging behind. It might seem ironic to be able to make something even though you don't understand it. But the fact of the matter is, I think cells, for the most part, know what they need to do. And very often they just need a little guidance from the outside or just the right environment to help them do what they have been programmed to do on their own. So a lot of the complexity that lies behind development, I think, remains to be discovered. And I find this really, really intriguing, coupled with the fact that we're now developing the tools to be able to understand them, like optogenetics, like, you know, we can actually advance this field forward. I think this is really an exciting area of research. It's also, you know, synthetic development is in particular, which, you know, crosses with synthetic biology is one such area, I think, that I would like to spend more time learning about and working on. As far as the future of synthetic biology in the whole, well, I think much has been written about the promise of synthetic biology. So there's no point for me to repeat all of that to you, if you all know it, if you're in the field. But my own perspective about how would one realize it? What needs to be done to realize this very bright promise of future? I think we have to be able, as a field, to tame the complexity of biology. We have to be able to reach a point where we can build reliable and predictable circuits. I mean, compare that, for example, to electronics. This is a dream, is to reach a state of electronics. So now if I want to build an electronic circuit, you know, I did this when I was an undergraduate and I did this as a hobby. You know, you want to build a particular circuit, AM, FM, radio or whatever, you design it, you go maybe to PSPICE, you simulate it, and you see that it works there. You go to the lab, you put the parts together. And it works the very first time, OK? This is where I think we need to be in synthetic. So, you know, really reliable, predictable circuits. And we're very far from that right now. I mean, there's a lot of things that have to be developed, a lot of things that have to happen before we get to this point, if we ever get to this point, I don't know. But I think regardless, we have to try. We have to have a more rational approach to building synthetic circuits. And I think what will take us there is a new theory for synthetic biology. So this is sorely needed. We need a new theory for synthetic biology when that is built around new design principles that are tailor made for synthetic biology, not borrowed from electrical engineering or any other fields, because at some level, I think the analogy between electric circuits and biological systems break apart. Modularity, for example, is one example. So we have to take stock of the fact that we're dealing with biological substrates that behave differently from electronic ones and develop design principles and concepts that are particularly suited for biology and accept the fact perhaps that, we're not going to have perfect modularity in synthetic biology, but then exploit that in our design. So we need design principles that are tailor made for synthetic biology and a theory around that. I feel that this is the way we will reach the goal of having more reliable and predictable circuits. And my own lab is working towards that goal. There are a lot of issues, a lot of problems that have to be solved, but I think that we just have to get started. Yeah, it's amazing to hear from an expert about the promise of synthetic biology and what does need to be done and standardize the parts and trying to make it close to the dream of electrical engineering and combining parts. So I think we are coming to our last question and the end of the talk. I will just ask a general question about the things that you would like to promote, DI efforts, research openings, papers, books, or anything you would like to add. OK, yeah, thank you for the opportunity to plug in some of our work. I mean, I would like maybe to promote two papers. So for the listeners who want to know more about this area that I call cyber genetics, I would propose an introductory paper. You don't really need any background in engineering or in biology for that matter. And the paper is called Cyber Genetics, Theory and Applications of Genetic Control Systems. And this has appeared in May 2022, issue of the Proceedings of the IEEE. I was fortunate enough to be an editor for this special issue on systems and synthetic biology. So I urge you to pick up this journal. It's not one that biologists usually look at. It's mostly for the members of IEEE, the Electrical Electronics Engineers Institute, which is one of the largest professional organizations, I think the largest professional organization in the world. But in that issue, we explore many aspects of synthetic biology, not just cyber genetics, but other aspects with articles, expert articles written by leading researchers in the field. So I urge you to take a look at that. And I would like to promote this here in terms of a more technical piece of work. I want to again, promote a paper that we have published recently in the October issue of PNAS last year. And this paper is about the title is Universal Structural Requirements for Maximal Robust Perfect Adaptation in Biomolecular Networks. And I'm really, really very excited about this work. In it, we try to essentially develop an internal model principle for biological integrators, biological networks, where we come up with simple conditions to characterize perfectly adapting networks using very simple algebraic conditions, just simply by looking at simple linear algebraic conditions. If you want something a little bit more, it gives you the more of a flavor about our control work on biology, but more technical than the first paper. But I think at least for me, it's very exciting, it would be that paper. Yeah, so those are those are the two things I would like to promote. Thank you for giving me the opportunity to do that. Thank you again, Mustafa, for joining us today. It's been a pleasure to talk to you and learn from you and hear about all these exciting research. And thanks, Ross, for co-hosting with me. Thank you, Mustafa, for coming on our show. Thank you, Rana, for co-hosting with me. This has been a pleasure. And that's it for the interview. So we'll have links in the episode description to the papers that Mustafa just talked about for our listeners to go ahead and dive right into. But we'll call it a wrap. So this has been another episode of EBRC in Translation, a production of the Engineering Biology Research Consortium Student and Postdoc Association. For more information about EBRC, visit our website at EBRC.org. If you're a student or postdoc and want to get involved with the EBRC Student and Postdoc Association, you can find our membership application linked in the episode description. A big thank you to the rest of the EBRC SPA podcast team, Catherine Brink, Fatima Enam, Andrew Hunt, Kevin Reed, Kokezi Li, David Mai, and Heidi Klumpe. Thanks also to EBRC for their support and to you, our listeners, for tuning in. We look forward to sharing our next episode with you soon.