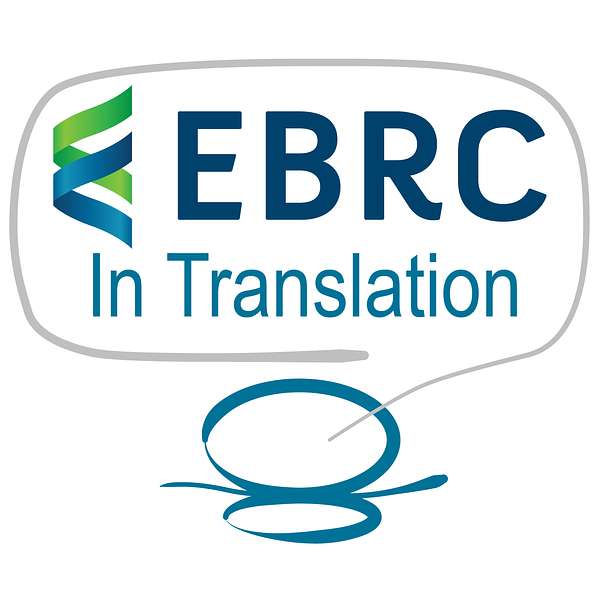
EBRC In Translation
EBRC In Translation
1. Drug Discovery and Corporate Coup D'états w/ Sri Kosuri
In this episode, we interview Dr. Sri Kosuri, an Associate Professor at UCLA and the founder and CEO of Octant Bio. We talk to Dr. Kosuri about his career trajectory, starting in the earliest days of synthetic biology up to the present, where his team is using next-generation DNA synthesis and sequencing technologies to accelerate the discovery of new drugs for the treatment of complex diseases. Along the way we talk about wikis before Wikipedia, engineering vegetables that are arbitrary shapes, and questioning our most basic scientific assumptions.
For more information about EBRC:
Visit our website at ebrc.org. If you are interested in getting involved with the EBRC Student and Postdoc Association, fill out a membership application for graduate students and postdocs or for undergraduates and join today!
Transcription:
Episode transcripts are the unedited output from Whisper and likely contain errors.
Hello and welcome to EBRC in Translation, a production of the Engineering Biology Research Consortium's Student and Postdoc Association. We're a group of graduate students and postdocs working to bring you conversations with members of the engineering biology community. We're your hosts for the episode, Andrew and Adam. I'm Andrew Hunt, a graduate student in synthetic biology at Northwestern in the Jewett Lab. And I'm Adam Silverman, also a graduate student in synthetic biology also at Northwestern and also in the Jewett Lab and also co-advised by Joyce Lux as well. And this episode, our guest is Sri Kishore from Octant Bio, a startup company working on using synthetic biology to accelerate drug discovery. Welcome to our show, Sri. We're excited to have you with us today. We'd like to start off our interview by asking our guests to introduce themselves. So can you tell us a little bit about your personal journey, starting with your PhD and kind of the early days of synthetic biology and ending up where you are now as the founder and CEO of a startup? Sure. First of all, thanks for having me on. This is really fun. I've been involved in the early days of SYNBURK, which was a predecessor to EBRC. And it's always been a great community for grad students and postdocs to meet each other and grow together in the community. And I think a lot of the people, including your advisors, Julius Lux, we met in the early days at meetings like this. So yeah, so background. My name is Sri. I am co-founder of a company called Octant on leave from UCLA. By way of background, I went to undergrad at Berkeley where I was working in engineering science and I saw a flyer that was posted by a new staff scientist at Lawrence Berkeley labs, who was trying to build like, basically seek volunteers to join the lab back when, I don't know, people flired the campus. I thought this was Adam Arkin. So Adam had flired the campus with this unintelligible flyer about stochastic gene expression. And I didn't understand a word of it as a freshman, but I took the flyer and I met with him and he was just like a force of nature at the time. And it really started my love of, I guess this was pre-synthetic biology per se, the term, but like just thinking, I think probably more in the systems biology world of thinking about cells and stochastic chemical systems that do some pretty amazing things and kind of started my love for biology. So I spent the first several years, about three years in that lab where I first met Drew Andy when he was at Berkeley and the molecular sciences Institute. So I was the first, I think at Berkeley, the first bioengineering major, the major started in my junior year. And I think there was no real curriculum. I think Adam Arkin just like made up classes I should take. And then I joined the nascent biological engineering department at MIT, first joining Doug Laufenberger's lab, but then Drew had moved in the middle of my first year to MIT as a fellow in the bio and bioengineering departments. And so I, at some point at the end of my first year, I switched into his lab. So then I was there. My undergraduate research apprentice for that first summer was Jason Kelly, who's now CEO of Ginkgo. He ended up joining the lab the next year, along with Barry Canton and others like Rachel Machete. My project was mostly initially on systems biology of a phage called T7. I was building these models kind of similar to what I was doing in Adam's lab, these stochastic simulations, trying to understand very simple systems. And even there, it was just too hard to model. We couldn't do experiments. We couldn't really do measurements. We couldn't do any of these things. I started a project basically to try to what we called refactor at the time, the virus genome. And so we were building basically synthetic viruses at the time that were more like our models rather than trying to be better modelers and make our models better. So that was like the early days. We started this thing called the synthetic biology working group, where we just kind of have homework and think about problems in synthetic biology, which were really fun things like iGEM and open wetware spun out of those things. I spent a bunch of my time on the open wetware stuff as well. We were trying to learn experimental biology in a vacuum and it's almost impossible, as you know. And so I was trying to get more of the tacit knowledge that people have directly onto the web when wikis were just starting. It was still a small community, like Jimmy Wales popped by one year during that time and I was just like, oh, another wiki, let's find out. And I think that was a really funny dinner because it was like us at the open, it was like me, Rachman, Jason, I think, and like Jimmy Wales. For some reason, I think Julius was there and the founders of Reddit. So like it was just a weird time in Cambridge. So let's see, this story is going long. I'll try to fast forward. We need this photo as like the Solvay conference of 2006 or something, right? So I graduated, I started a postdoc in Mike Laube's lab, mostly because I think I played Frisbee. We were in this league, this Boston league, Ultimate League. Mike Laube, if you don't know, is a professor at MIT, HHMI, like does amazing work on cell cycle and colobacter and two component systems. But I knew him from Stanford. So my project at Berkeley was this project with Dale Kaiser, who worked on this bacteria called Myxococcus anthus. And he was across the hall in Lucy Shapiro's lab. And so I had met him a few times. He moved to Harvard as a junior fellow or these kind of Bauer fellows, and then moved to MIT to start his lab. I met him socially a few times playing Frisbee. And so I was like, Oh, well, this is a cool lab. Let me join. So like, kind of the serendipity happened over and over again. But within the first six months, I kind of, I've always been really applied and was like, really interested in how we engineer biological systems that do stuff more than just discover science, but like to build things that, I don't know, kind of nudge the world towards other things. And I think at the time I was like, here are like five ideas that I would go into industry for. And one was like algae to fuel. This was when gas prices were high. One was in vitro meat, synthetic materials. This is kind of like a bunch of these kind of companies started around that time. And a classmate or friend of mine at the time, this guy, David Berry, had just joined flagship ventures. And he was starting a company out of that because they had been spinning out companies. This is before they have this thing called venture labs now, but at the time they were spinning out this company. It was like, Hey, do you want to think about this with me and help start it? Right. Like, and so at some point I was like, yes, that's the most exciting thing I want to be doing. So I left pretty early in the postdoc, about six months in and went to flagship and then became the first employee of a company called jewel. We can dive into this later, but it was really fun and really hard. I was fired maybe two years, almost two years in in an attempted coup that didn't work. I don't know how to describe it. George is the head of the scientific advisory board and he's like, Oh yeah, you're probably going to get fired for doing this. You should come join my lab if you do. And so that's George's lab. Yeah. So I worked there on DNA synthesis technologies. We spun on a company called gen nine out of some of that work, worked on an editing technology. So like my benchmate was Fung Jung, who like we were working on talents at the time. And then it felt too competitive for me. And then I started really working on the things that turned into a lot of what I started doing at UCLA and elsewhere, which were kind of like, we could make lots of stuff with synthesis. We can put them into cells with editing, but then you still had to do the experiment, which took a long time. And then, so a lot of what I started working on it, how do we like multiplex experiments? How do we do many, many experiments at once where one person at the bench can do that? And a lot of that turned into like, how do we convert as many assays as possible into ones that can be read by next gen sequencing? So then that started in bacteria, I started a faculty position at UCLA. We worked at a bunch of things like DNA storage, transcription, translation of bacteria, then in yeast, looking at complex traits. But it was also around the time that a lot of my interests changed. Like I started having kids, I started like, instead of going from like, I want to build like cool designer pets, to turned into like, there are things that we can do in human medicine, and I want to learn more about it and try to point my career that way over time. So a lot of what happened at UCLA was, how do we take these tools, which I felt finally felt complete, right? We could build and test things at scales that like I dreamed about in the early days. And so we did a bunch of different types of things. But, you know, I think the more I understood things around genetic variation and how it impacts disease, and, you know, my imagination of what therapies would look like, we're like, we're going to go fix all the mutations that are bad, right? And it turns out for most diseases, most complex diseases, that's not going to work. And so a lot of the thoughts around like, are there angles to take that aren't being really done right now, that we could take these types of systems to help deconvolve this complexity and build more rational approaches in these areas. So that's that's kind of what started Octin. And so here we are today. Well, very, very cool. You've touched so many different areas, and it's really neat to hear about it. So thanks for sharing all that. So on the subject of octant, maybe you can give us a little more insight. You sort of briefly touched on it. But can you talk a little bit more about what the problem is more explicitly that you found an octant to solve and what exactly you all are doing to go about that? Yeah. So maybe just pick up where I left off, you know, a lot of what we're doing in early days in my lab, we're like tracking down causal variants in looking at complex traits. And we we published something recently in autism on like finding causal variants in like basically enhancer regions, we've done stuff on splicing, looking at rare variation. A lot of these things were interesting. I think there is a world where we start moving towards understanding which of these variants are causal. But the end result of that felt very lacking for me, which was like, okay, at the end of the day, what this is saying is that large numbers of small effect variants kind of accumulate, because they're all super small effect in the common variant realm accumulate in complex diseases to drive a process into pathology. And fixing that is super hard. Like you can't just go change all those variants. There are good drug targets, they tend to be the central nodes in physiology. So basically something that like affects many downstream pathways, and they tend to be really dirty. So there's tons of what's called like on target toxicity. So the same thing that you're trying to target in one cell type does something completely different in another cell type, for instance. And so how do you fix that, right? Like, it's not like you can make it more specific, because it tends to be, it tends to be the same exact protein. And a lot of this started from work in our lab around key protein coupled receptors. So GPCR is like, you know, there's about 800 of them in the human genome, half of which are responsible for taste and smell, but the other half responsible for like tons of central processes in almost every vertical in pharma. Like the top 50 or so are the targets of about a third of all FDA approved drugs and indirectly, and about 50% of prescriptions. So huge drug class, we know they're physiologically relevant, you modulate them, you modulate phenotypes. The problems with them is that A, very good drugs, like one of the receptors we studied was this thing called beta two adrenergic receptor, which is a classic receptor in this class. It sits in the smooth muscle cells of your lungs, you agonize it, it contracts those muscles, so it opens up your airways. It's going to be super hard to find another target that does that well, right? Because it coordinates the setup, like basically a signal transduction cascade set that affects many genes. And B, the problem with the target is, it's a great target. It works, right? Like if you get a smooth muscle cell in your dish, and you like, you're just going to get a beta two adrenergic receptor agonist. But the problem is that that same receptor exists in your heart, controls heartbeat, targeted beta blockers, same exact protein in a different part of your body, right? Like in your brain, we don't know actually what it does, it's all over your brain, but like it controls parkin expression. So people that take albuterol tend to have 2x lower Parkinson's disease risk, right? So that's like, what do you do? And the long and the short of it is that if you look at the best in class drugs for a lot of complex diseases, in fact, the most complex diseases, they tend to be super dirty. And they tend to hit lots of GPCRs. And so there's a lot of what's called polypharmacy. But not only that, you can also hit multiple pathways from a GPCR and certain ligands hit some pathways stronger than others. And so that's called functional selectivity or bias. And so what people have been seeing is A, like a ton of drugs already in existence have a lot of uncharacterized polypharmacy and bias and kind of the profiles of side effects and things like that are, are there better ways to think about those profiles? But the bigger point is that the secular trend in pharma over the last like 50 years has been about specificity, right? It's a bit taking these kinds of drugs and making them ever more specific, whether that be like structure based drug design or, you know, all the way to AI for drug discovery, but even things like ASOs, antibodies, things like that, all about specificity. Everything about complex traits tells us we're not going to find a magical target in these spaces for complex diseases. You know, maybe in some cases, but like there's a, there's a pretty good chance that that's not going to be the case, right? And so the question becomes like, how do you achieve specificity in these types of disease classes when the targets aren't specific, right? And, and the answer to that is there might be many things. Maybe it's like gene therapy in the cell types that you want. But another way to think about that is that if you're in a lot of complex diseases, it's like some process or cell type that's going wrong. Think like beta cells and diabetes or certain subsets of neurons and neuropsychiatric diseases. If your target is actually the change in physiology of the cell, each cell expresses, you know, 10 to 30 of these GPCRs and communicate through the same pathways. So can you achieve specificity on things like cell type rather than on a particular receptor itself? And if you like change that viewpoint, all of a sudden you, you can develop rational ways to think about them because of things like single cell sequencing, because of large scale genetic acids, we can start narrowing down what the rational kind of vectors of polypharmacy and bias we should start thinking about. So like the company is really split in two parts. One is like, how do we uncover those things? A, how do we use large scale kind of the engineering of biology to uncover the complexity of these target profiles? And then B, if we can do that, how do we build small molecules or molecules in general to go and go after those kind of complex targets of physiology? And so that's how the company is split up right now. And that's like probably the biggest bet we're taking at the company. Very cool. So I guess maybe following up with that, the key enabling technologies that set Octant apart from, you know, rational structure-based drug design, what would you say is different from an experimental perspective that's enabled you to really make these plays? Yeah, so technologically, the foundation is really probably three sets of technologies, two of which are highly related. One is like, we engineer cells to act as good reporters of physiology, right? And what we can do is instead of using like GFP or luciferase assay to like read that out, we do that through barcoded experiments that allow us to multiplex. That takes like two forms at the company. One is this kind of deep scanning mutagenesis, which is kind of becoming more and more routine in the field, which is like this ability to make all possible mutations to a particular gene. We just published this week, I think it came out in eLife around the beta-2 adrenergic receptor deep scanning mutagenesis, where we make every possible mutation to this receptor in human cells and then read out functional activity. What we show in that paper is that that's A, useful for interpreting large-scale genetics and biobank data. And that's interesting in figuring out if it's a good target or not. But like B, starts giving you structural information, where classically structure is hard in GPCRs because they're like a pretty floppy protein, very dynamic, cause differences in signaling pathways. A snapshot of structure, which is already hard to do, like it's hard to get a native structure of GPCR, doesn't get you as much information as one would hope. So what we show in that paper and what we've done subsequently at Octin is like using that as a way to probe structure function in a process called basically structure-activity relationships, where we can run a series of ligands to understand how different residues are contacted based on the constraint to mutation that those residues see. And so we basically think about deep scanning as a way to do those two processes, interpreting genetic variation, but B, building small molecules that basically drive things like polypharmacy and bias in more rational ways. The other kind of thing that we do with the barcoding is in a single well of a 384-well plate, we kind of optimize this in a way that lets us do like 100 or so barcodes at the same time. And then we can run that like 10,000 wells at a time or so. Right. And that's done by like, you know, one or two people in a semi-automated way. And so we can just like turn that. We basically run that every week at this point. And the scientists at Octin use that as a way to A, like optimize the synthetic biology. So how do I build a reporter to receptor blah, right? Whatever it is. Well, I can try like hundreds of different designs and that goes through our platform and we optimize that on the platform itself. And then once it's optimized for the library we want, we look at ideas of polypharmacy and bias in order to one, map existing chemical matter onto those like kind of complex relationships where polypharmacy is most manifest. And then B, we use it as a way to build new drugs with designed ones. And so that is a third leg of the technology, which is like a high throughput synthetic chemistry capability that allows us to do essentially fragment-based drug design at scales that are similar, like five to 10,000 molecules at a time, but quickly. So it just lets us turn that process way faster between design experiment. So those are the kinds of three major technologies and then like the bets of the company sit on top of those technologies. Awesome. Thank you. That was very informative. I want to maybe take a step back from the technical questions and maybe more directed on the company itself. So like you alluded to, you were part of the founding of maybe some of the earliest synthetic biology, depending on how you would define that companies with Juul, with Gen 9. Maybe those experiences didn't end quite the way that you wanted, but I wonder if you can talk a little bit about what it taught you about founding a successful startup and maybe if that's impacted how you think Octant should structure. Yeah, you know, we're in this golden age of lots of capitals, chasing not as many ideas. And so like that always wasn't the case, right? I think the idea of founder driven biotech. So I remember like I was starting Juul through this kind of VC route. And even that was kind of crazy, like that, like a bunch of PhD students out of a VC firm could start companies. And so flagship was really pioneering that. But then like, you know, Ginkgo and Jason and all those groups, that group was just like, oh, we're just going to start our own company. I was like, you guys are nuts, right? Like, no one does that. And, you know, like, I think culturally, so I think there's a couple of things. So I don't have any problem with the models that like most biotech VCs do now, which is like, create companies, bring in strong leadership at senior levels, after doing some early de-risking experiments with young folks, and then build that company in a way that usually gets sold as a way to do like externalized innovation. Totally get it makes sense. But I think those companies, it's really hard to get the culture right, right? Like, is this a place that you want to work? Is this a place that's actually going to do really new stuff? I think there have been examples where it's worked. I think it's really, really difficult, right? Because like, it's hard to take a bunch of senior leadership and install it in a company and try to actually build culture in a way that makes sense. So like, I think for me, after that jewel experience, I was just like, I'm not going to do a startup that's this way. And I don't even think I'm going to go back to startups. Like, I'll sit on SABs and it'll be fine. And so that's what I did with Gen 9, which is more like at the SAB level, kind of helping, you know, start some of the technological stuff, but let them go and figure out the business. So fast forward to UCLA, I think that what changed for me is one, like, there was just a lot more capital backing earlier people. Like, I think places like Ginkgo and others like showed that founder driven biotechs can do things that are outsized. And I think, I think people forget this, but that was hard to do in tech too, like before Facebook and Google and like, you know, like before those successes, tech was exactly like biotech. So I think the thing that changed for me is a couple of things. One, I felt like that cycle, that whole cycle of design, build and testing, basically just all like 100x at the same time. And so that was finally coming together in a way that was possible that individuals, small numbers of people could do these experiments that no one could, like one grad student, Eric, who's now at Octon and as an early employee, but like, you know, made every possible mutation in human cell lines to a receptor and like that's crazy. Like it involves like large scale DNA synthesis, large scale editing, large scale, like measurement all at the same time in a single dish in a way that not even like a big pharma could do individually with as much automation as you wanted. Right. And so like what happened technologically was this, this convergence where individuals could do these magical experiments that never were possible before. And I think the thing that changes then is that those individuals are all super young. Like it's not like, it's not like it's going to be easy to install like senior leadership in this place, mostly because like none of those people have any intuition as to what's going to be feasible and what's not. What's like a good idea and what's not, right? Like I think the people that actually live and breed that stuff are the best place to understand that. I think that's one. I think two, like, I am good at some things, but also bad at lots of things. Like, and I think for me, one of the amazing things about academia is you can work on all sorts of things. You get to train people. It's a really fun environment. You get kind of refreshes of people and you get to kind of try new things. But I'd say like one of the things about being a PI that people don't talk about a lot, but if you talk to a lot of young PIs is that it's a really lonely experience. You've spent your whole career in these labs that are super collaborative and fun and like you make fun of your PI together, right? And then all of a sudden you're the PI and it's just like, there's a power structure in a way that you can't be like one of the gang like you were and other PIs don't really have time for you. And they're all like too busy with their science and that makes sense. And so like, and then in building a company, there's like a lot of parts of running a company that are really hard. And so long story short, like I had a co, like a friend, a family friend that I've known for a very long time was early at Google and then Dropbox and then things like that. He had like, not retired, but like kind of was like, look, I was a bioengineer undergrad. I want to like kind of think about what's next. I have had this successful career in tech and he's built like tons of teams, you know, from like dozens to thousands of people at companies and like understands like how to build culture and growth and like high performance teams. And so like, I think we were talking and a lot, at some point we're just like, let's just do this. Let's just see what happens. We're all like, life is short. We get a certain number of bets. Can we build something culturally that we want to spend our time on, on problems and no one else is really attacking. I think a big portion for me was like, yes, we could be the like 30th CIN biocompany working on like designer gene therapies, right? But how much extra Delta are we actually going to provide? And probably the answer to that, it was like very little. And I think for us, it was like, can we ask and answer really big, tough bets with a great group of people with a culture that is about bringing science and scientists to the forefront of those and like giving them increased responsibility. I think one of the things people find in startups and you'll hear this at other places is like, the only way to move up is to, is to leave and join another startup, right? Because people will just hire over you. Other things you hear is like, oh yeah, the only way to like grow with the company is become a manager rather than a scientist. And I think there are ways to do that. And tech has done this in ways I think that are really interesting. And I think we can bring a lot of those to biotech. And the other thing is like, for us culturally, it was like, there's all this stuff going on in CIN bio in companies and like, it's super cool. And like, can we build a culture that's more collaborative around, you know, no one's going to do the stuff we're doing, honestly, right? But like, the challenge before us over the next like 50 years as a field is so big that we should just be building a culture that's helping each other out. And can we like be a part of that change as well, both from industry and from academia. So I think, I think it just felt like the right thing to do. And that's a very strong part of who we are. And so like, I think the moment that stops, I think, like a lot of the dream is dead in some ways. But like, until then, it's been fun to do. Very neat. That sounds makes me want to be a part of a company much more so than maybe I did before, at least you've convinced Andrew. So you've spent a lot of your career also looking at and focusing on enabling technologies for high throughput DNA synthesis. Can you talk a little bit about what you think maybe current technical gaps are in the field for maybe cheaper and more scalable synthesis? And what do you think those advances will ultimately enable? Yeah, so let's break down the question a little. So like, I think DNA synthesis is incredibly powerful. I spent a large part of my career trying to focus on it. I think the reason why I think this is that, I mean, I think the simplest analogy is biology is this crazy alien technology that can do things that we can only dream of, right. And we're nowhere close in understanding it despite our like, you know, like what we talk about and how we talk about it. Like, there is a deepness there that is profound. And I also think we don't even understand the language by which it's able to do what it does, right. Like, we think of these things as parts. And like, I think all of that is like, I don't know, I'm not a big proponent, because I think the power of biology comes from the ability to do logic and things without having all these like kind of separations. And I get the microprocessor analogies. But I think what I want to be able to do from, you know, a technology standpoint, it's like programming its own language and not try to understand. And so one way to do that is to like build up this ground model, physical model, you know, I understand the physics, I understand the chemistry, understand the biology, understand the function, I understand the phenotype. The other way to do that is like, treat the cell as a physical model, and the inputs are DNA, and the outputs are like the function or phenotype. And if I can like, do that input output really broadly, I might be able to better at least get a grasp on how to do that. And so part of that is DNA synthesis, part of that is measurement. And I think they're they're fundamentally linked. But when you can do both, that's amazing. That's how I think of it as a way to uncover complexity in these kinds of systems, and to build physical models of biological processes. So from a technological standpoint, where do I see the field going? Like, look, I mean, in the T7 work, we you know, there were many predecessors to Gen 9. But when I was first working on it, it was like, super expensive, it was like $4 a base. But then when I talked to George Church is like, look at my first oligo order was like $4,000 a base, right? So like, but you know, we paid for a 4kb thing that didn't end up being useful because it failed. But it was like a 4kb times like $4, it was like $16,000 or something. Now, like, you know, 4kb is like a few hundred bucks. So like, there has been massive improvement, even in the timescale I'm talking about, which is like 10 to 15 years. And that's a pretty big drop, but it's not like this drop in sequencing. My fundamental belief here is that I don't think there's anything technological stopping us from getting low cost synthesis going. There's like 100 different ideas that I think have promise and should probably invest be invested in if, if you just view it from a humanity, like, we should be spending money on this and not necessarily on other things. But do I think that'll happen? And I think my answer to that is probably not. There's not really great business models for DNA synthesis. I think twist has been uniquely forcefully like amazing in this, but even they will have I think so just to give you some example, like, what drove sequencing was this dream and expectation that we're going to sequence everybody in the planet, right? So 7 billion people, that's a huge market. The problem with synthesis is that there's like, a very tiny fraction of those 7 billion people are scientists that might make DNA. And a much tinier fraction are like synthetic biologists that actually consume DNA. And like when I went around working on DNA synthesis, my biggest problem half the time was like trying to find things to design, right? So I'd go around like I went around to MIT and Harvard around the time where I was doing some of the first stuff that led to basically the technology that Gen 9 was doing. And I was just trying to find reasons to build stuff. And I'd go around and I went to Jim Collins and all these people. And like, and everyone would be like, yes, I have like, I need DNA synthesis. I need a ton of it. Let me get back to you in like a week or two or three or whatever go by. And it would be like, I need these four 2KB constructs. And like, and like, at some point, it started as a joke where I was like, George, can I just build like Moby Dick and DNA? And like, he's like, no, you need to build something useful. Nathaniel Hawthorne, not Herman Melville, right? Everyone like I went to like, and unfortunately, like, I don't know if you heard, but I'm Angelica Amand and MIT passed away yesterday. And she was like a force of nature. And just I really loved her work and just admired her. And I went to her and I was like, what do you want to build? And I'm like, she's like, I want to build a yeast chromosome. Like, oh, that seems cool. Like, why? And she's like, I want to build it without like any proteins on it. And I'm like, that's weird. I want it as a control experiment to this thing. And so I'm like, you want me to attempt the largest synthetic biology project ever thought done for your control. And it's just like, it's just like hard to get people weren't thinking design wise on that scale. And we ended up doing like the DNA book project. We didn't do Moby Dick because we were worried about copyright because Venter had just published this like quote from somebody in some organism and like, their estate of that family like complained or something. So we're like, okay, we're just going to do this book. But like, that DNA, since the book project was like, that is only like large scale consumer level use of DNA synthesis that we could think of broadly that would drive synthetic. Like, the reason why we did that project was to like, give people like a vision of like, hey, maybe DNA synthesis could be a large scale thing. But otherwise, absent that, it's a research tool, it's going to get the investment that research tools tend to get, which are small. And no one's really willing to pay up for the other side of it, which is like, this enables a bunch of other stuff, but no one's going to give you that downstream value. So it's not going to drive a lot of private investment. And so like DNA synthesis is a business model, something I vowed to not like ever do again. I think that might be changing. And hopefully it'll change. But people say people have been saying that for like 20 years, right. And it's always comes down to like, the experiment is the limiting thing. Like one of the biggest, you know, maybe like some of the biggest customers in time of jet nine was like places like Monsanto, they're like, I don't care what I'm paying for this, like 100 or $200 DNA synthesis thing. The experiment I have to do is going to cost me $100,000. So just make sure it's perfect and like, get it to me. Right. And so like, I guess the point is, is that DNA synthesis is already cheap enough for most applications, because everything else is more expensive. And it doesn't do you any good to make it any cheaper because your experiments still cost more. And so I think if you want DNA synthesis costs to go down, you have to like up the demand side, which is like you need experiments that are way, way cheaper. That's really interesting. And I don't think I've ever quite framed it that way. I'm curious how you think about something like DNA data storage. Do you think something like that could be a significant driver in this area? Um, you know, like, we worked on some of the early stuff, you know, that was a really fun experiment. In the sense that like, we had designed, like I had designed this project, I wasn't really planning on working on it. And then at some point, George wanted to get back into the lab. And I'm like, I have this perfect project. It was like, so he cleared out like two weeks of time, like from the schedule and like use my bench and was like, doing these experiments and partially like, you know, I'd set it up for him. And he was blown away. It's like real time PCR. You can watch your PCR happen. And so, and so anyway, so he ended up like being the first author on the paper and I was the last one. So we like switch roles for this work. But you know, it was one of these things where it was just kind of like this can happen. In theory, it makes sense. It's not a terrible idea. If we think about what a new chip or a new nan memory, nan memory, like production platform or looks like, or facility, it's like billions of dollars. Like think about how much money gets spent on this stuff. Is there any kind of investment like that for DNA? No. The general idea of polymer storage, I think is a very good one. And it could take off if there were like similar types of investments. My guess is if it ever, if that ever happened, I think it would be super interesting. But my guess is the polymer that would end up winning is not going to be DNA. Right? You'd build polymers that were more optimized for that purpose. And in my mind, the reason why I stopped working on it was like, I love biology. I don't care about storage. Like, it's just like more came down to like, what do I want to spend my time on? But I do think, look, I think it's interesting. Do I think it's more interesting than like positioning xenon atoms on like in a four degrees Kelvin in a vacuum? Yes. I think it's more like, and there's huge amounts of resources, like 100x more resources put on those problems. And so yeah, I think it's super interesting. I just think it's a storage problem, not a biology problem. And I think could it drive more investment in DNA synthesis? Yes. And that's already happened, right? Like there are plenty of companies right now in stealth, some not in stealth that are doing that. I'm excited, right? I hope it has spillover effects in biology, but I care about the biology in the end. Yeah, I think I ultimately do myself as well. But it's cool. I hope it works. I'm very encouraging to the field, but it's hard, man. Storage is cheap. It's really hard to build companies when you're competing against like commodities, right? And especially storage, which is improving super fast. And so like that's a hard nut to crack and like Godspeed to the people trying to do it. Like I hope for the best. I'll ask this. I think it's a great follow up to what you just said. If you had to take a step back and there's a biological question that you are really interested in studying and you had six years of a PhD again to do the lab work using the DNA synthesis and sequencing technology that we have now in 2020, what would be the problem you would really just love to study? Yeah, OK. So like, I love this question because I constantly think about it. And like, you know, there are sad parts about leaving academia and it's this, right? Is that like you don't get to scratch those itches. So what are the things? So like the way I reframe this question in some ways is like, what are the things I would work on? Like if I were still in academia or starting as a grad student? Yeah. So I think if there's any history that I'd like to look at is like you want to jump onto things that are like exponentially improving. It's still a preprint. I don't think we've even submitted it yet on like so Guillaume Urtecho was a grad student in my lab, worked on a nice little system to look at bacterial promoters. Right. And the cool part about that is like he can train an undergrad to run that experiment, like a hundred thousand promoters at a time with complete synthetic control and a very simple experiment. Right. And just turn that crank. Right. So all of a sudden you have a system where you can make a hundred thousand physical systems and measure them with complete design capability. And it's like, yeah, who cares is bacterial promoters. Right. But like, I think it's like fascinating. Like, why can't we know if a sequence is a bacterial promoter or not? We don't even know the answer to that question for E. coli in the best, most well-understood molecular biology experiment in the world. Right. Like I think that is like ridiculous. What are we doing if we can't even do that? Right. Like so the question is given a hundred thousand experiments on, I don't know, every two weeks, what would you do? How would you think about it? Right. This in my mind is like, Hey, there's no other analogy to it in any other physical system. Like you can't make a hundred thousand robots. You can't make a hundred thousand like materials. Right. Like we can do this week in week out for cheap. So buy an undergrad. And so like the question is, is like what we would do. And so like in my mind, there's like a bunch of, there's like things like active learning. Right. So like this ability to take kind of deep learning mechanisms and then to do the design of the experiment. There's things like, let's just take the scientific method on, make it on crack. Right. Like let's just take every idea that I can think of, parameterize the models, fit it on the thing, and then build the sequences that most differentiate those models. Right. So that's like invalidating hypotheses, but on a systematic scale, there's learning represents like kernel methods that allow you to estimate, estimate uncertainty in these things. Let's build the most uncertain sequences over and over and over again, until we just achieve some broad metaphor, but like still, this is like a hundred and 120, a hundred, just say a hundred bases long, four to the hundredth is what two to the 200th, which is 10 to the 80th somewhere around there, whatever 70th, 80th. It's like way bigger than you're ever like a hundred thousand is to the fifth. Right. So you're never going to explore all of it. You're never going to even compute all of it. Like, right. Even if you had like a super fast, even if it took like one floating point operation, you'd never compute all of it. Right. In the, in the time of the universe. So like, the question is, is like, how do we build a model of that kind of complexity and how do we like build certainty in that much space? I think that's a fascinating question. And like, we finally have the tools to go do that. So I think that's one. I think the two would be, I want to come back. I'm like dreaming of coming back. Like my first paper, although it was published after my T7 refactoring paper was on building computational models of physical systems. But like, I think there's still fundamental questions in like simple system, like bacteria. We have genome wide measurements of like, you know, ChIP-C, RNAC. We can do all of these genome wide measurements, but we have no like theoretical or theory way to like interpret, does this all make sense? How many polymerases do they have? Where are they expected to be on the genome? Does that make sense with what shows up in RNAC? What are we missing? I think we don't have like a formal system by which to like explore those questions. And I think I would go back to simulation in some ways as a way for us to like explicitly put down the set of assumptions that we just kind of assume and ask from a step by step basis, like, do our assumptions lead to the things that we measure? Right. But the thing that's changed is that like we can do, we can measure and manipulate those things in ways that let us like constrain those models in a way that never existed 20 years ago. I think people are starting to do both kinds of things in the protein design space, which I think is exciting. But like, I think there's just like fairly simple questions in a bunch of the stuff that we can like attack now. So in my mind, a lot of those would go back to original questions.